Mishal Fatima
Paper download is intended for registered attendees only, and is
subjected to the IEEE Copyright Policy. Any other use is strongly forbidden.
Papers from this author
Global Feature Aggregation for Accident Anticipation
Mishal Fatima, Umar Karim Khan, Chong Min Kyung
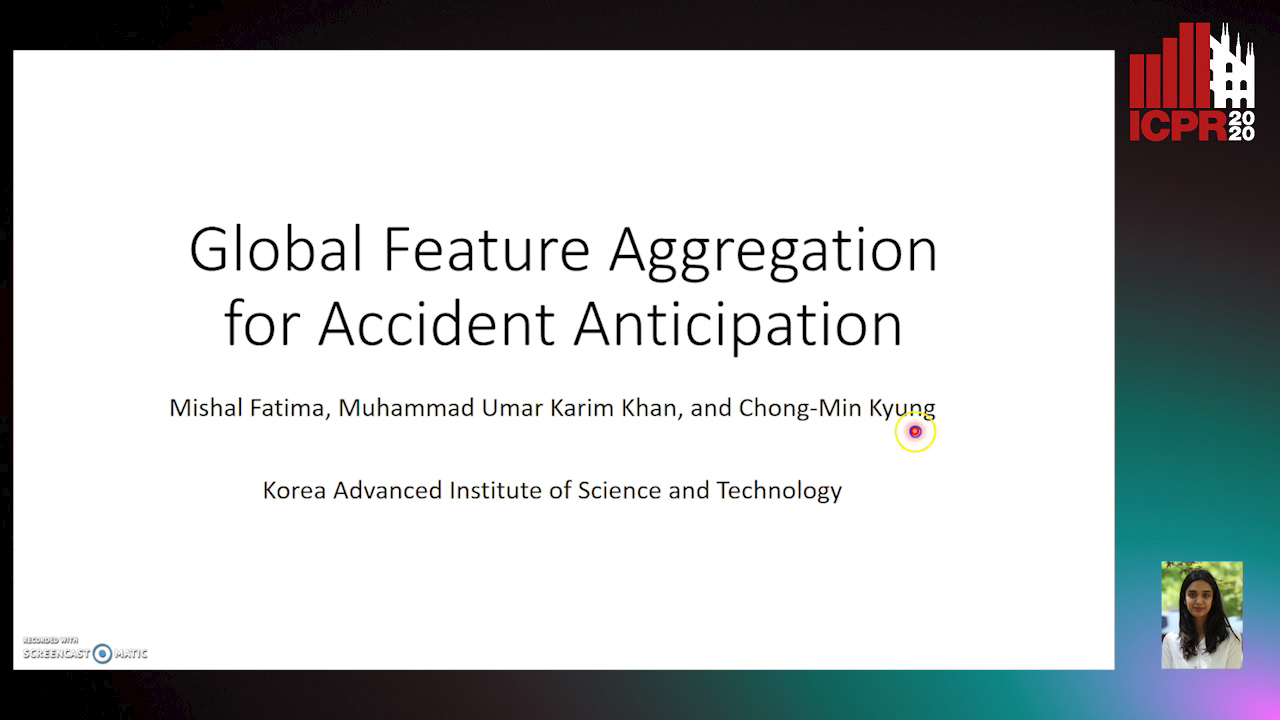
Auto-TLDR; Feature Aggregation for Predicting Accidents in Video Sequences
Anticipation of accidents ahead of time in autonomous and non-autonomous vehicles aids in accident avoidance. In order to recognize abnormal events such as traffic accidents in a video sequence, it is important that the network takes into account interactions of objects in a given frame. We propose a novel Feature Aggregation (FA) block that refines each object's features by computing a weighted sum of the features of all objects in a frame. We use FA block along with Long Short Term Memory (LSTM) network to anticipate accidents in the video sequences. We report mean Average Precision (mAP) and Average Time-to-Accident (ATTA) on Street Accident (SA) dataset. Our proposed method achieves the highest score for risk anticipation by predicting accidents 0.32 sec and 0.75 sec earlier compared to the best results with Adaptive Loss and dynamic parameter prediction based methods respectively.