Dmitri Jarnikov
Paper download is intended for registered attendees only, and is
subjected to the IEEE Copyright Policy. Any other use is strongly forbidden.
Papers from this author
Video Representation Fusion Network For Multi-Label Movie Genre Classification
Tianyu Bi, Dmitri Jarnikov, Johan Lukkien
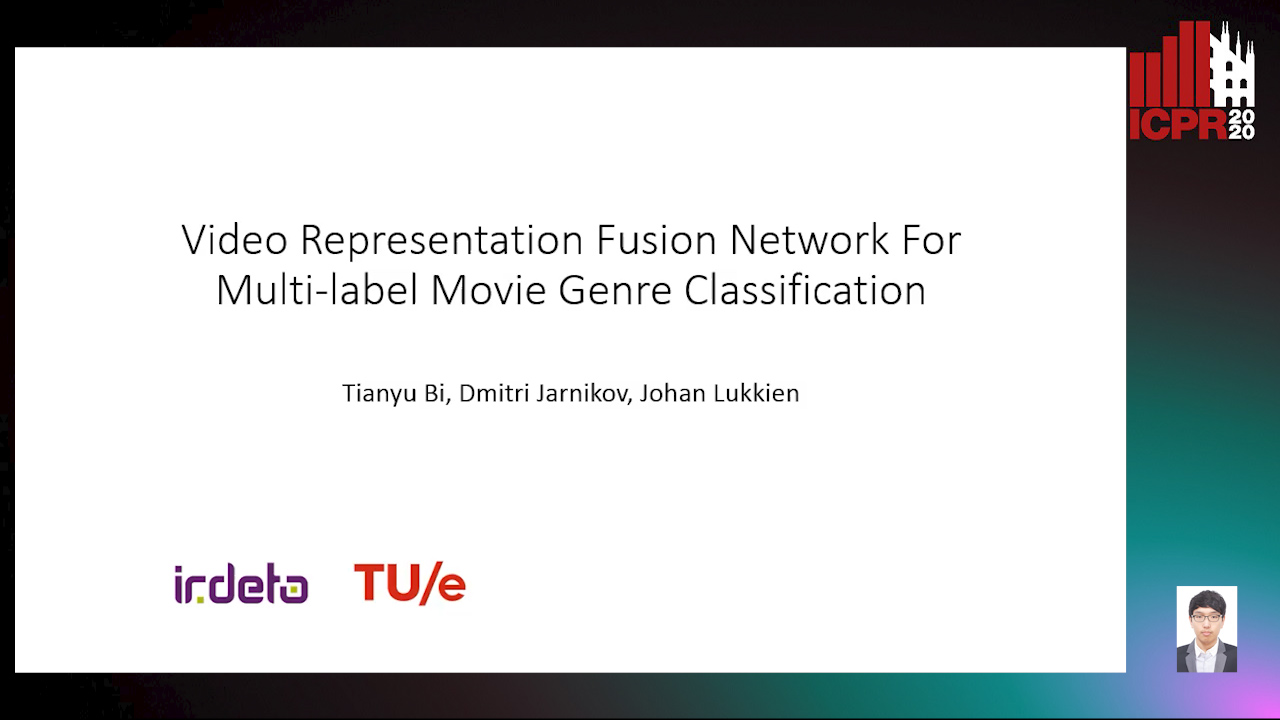
Auto-TLDR; A Video Representation Fusion Network for Movie Genre Classification
Abstract Slides Poster Similar
In this paper, we introduce a Video Representation Fusion Network (VRFN) for movie genre classification. Different from the previous works, which use frame-level features for movie genre classification, our approach uses video classification architecture to create video-level features from a group of frames and fuse these features temporally to learn long-term spatiotemporal information for the movie genre classification task. We use a pre-trained I3D model to generate intermediate video representations and connect it with a C3D-LSTM model for feature fusion and movie genre classification. LMTD-9 dataset which contains 4007 trailers multi-labeled with 9 movie genres is used for training and evaluation of the model. The experimental results demonstrate that learning long-term temporal dependencies by fusing video representations improves the performance in movie genre classification. Our best model outperforms the state-of-the-art methods by 3.4% improvement in AUPRC (macro).