Patricia Devlin - Hill
Paper download is intended for registered attendees only, and is
subjected to the IEEE Copyright Policy. Any other use is strongly forbidden.
Papers from this author
Multi-Level Deep Learning Vehicle Re-Identification Using Ranked-Based Loss Functions
Eleni Kamenou, Jesus Martinez-Del-Rincon, Paul Miller, Patricia Devlin - Hill
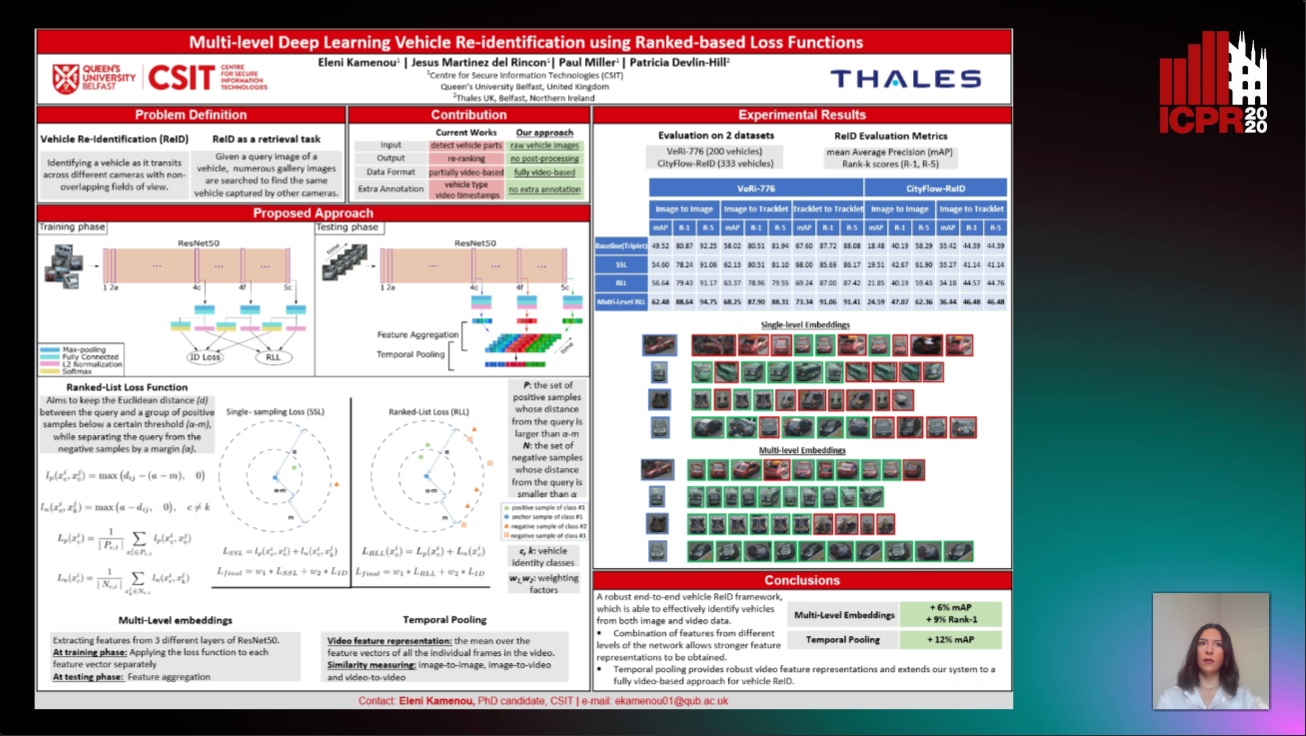
Auto-TLDR; Multi-Level Re-identification Network for Vehicle Re-Identification
Abstract Slides Poster Similar
Identifying vehicles across a network of cameras with non-overlapping fields of view remains a challenging research problem due to scene occlusions, significant inter-class similarity and intra-class variability. In this paper, we propose an end-to-end multi-level re-identification network that is capable of successfully projecting same identity vehicles closer to one another in the embedding space, compared to vehicles of different identities. Robust feature representations are obtained by combining features at multiple levels of the network. As for the learning process, we employ a recent state-of-the-art structured metric learning loss function previously applied to other retrieval problems and adjust it to the vehicle re-identification task. Furthermore, we explore the cases of image-to-image, image-to-video and video-to-video similarity metric. Finally, we evaluate our system and achieve great performance on two large-scale publicly available datasets, CityFlow-ReID and VeRi-776. Compared to most existing state-of-art approaches, our approach is simpler and more straightforward, utilizing only identity-level annotations, while avoiding post-processing the ranking results (re-ranking) at the testing phase.