Shuheng Lin
Paper download is intended for registered attendees only, and is
subjected to the IEEE Copyright Policy. Any other use is strongly forbidden.
Papers from this author
Dual-Mode Iterative Denoiser: Tackling the Weak Label for Anomaly Detection
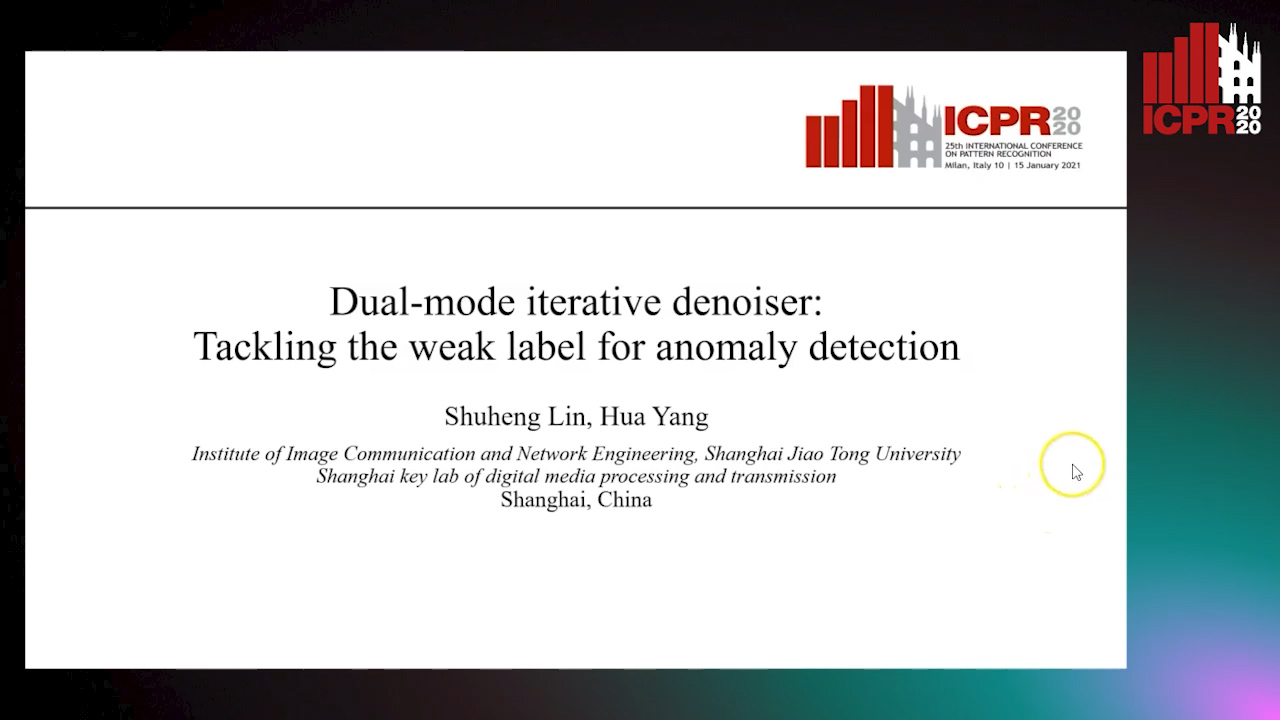
Auto-TLDR; A Dual-Mode Iterative Denoiser for Crowd Anomaly Detection
Abstract Slides Poster Similar
Crowd anomaly detection suffers from limited training data under weak supervision. In this paper, we propose a dual-mode iterative denoiser to tackle the weak label challenge for anomaly detection. First, we use a convolution autoencoder (CAE) in image space to act as a cluster for grouping similar video clips, where the spatial-temporal similarity helps the cluster metric to represent the reconstruction error. Then we use the graph convolution neural network (GCN) to explore the temporal correlation and the feature similarity between video clips within different rough labels, where the classifier can be constantly updated in the label denoising process. Without specific image-level labels, our model can predict the clip-level anomaly probabilities for videos. Extensive experiment results on two public datasets show that our approach performs favorably against the state-of-the-art methods.