Yiqun Li
Paper download is intended for registered attendees only, and is
subjected to the IEEE Copyright Policy. Any other use is strongly forbidden.
Papers from this author
Rethinking of Deep Models Parameters with Respect to Data Distribution
Shitala Prasad, Dongyun Lin, Yiqun Li, Sheng Dong, Zaw Min Oo
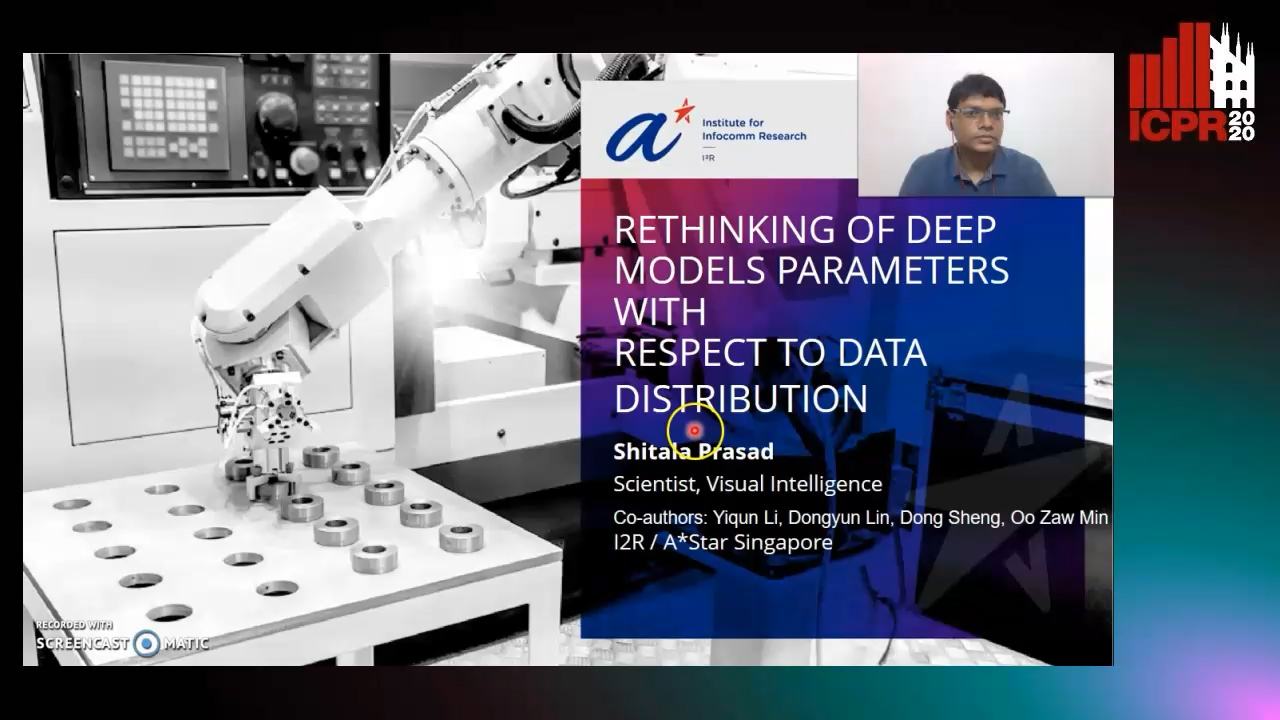
Auto-TLDR; A progressive stepwise training strategy for deep neural networks
Abstract Slides Poster Similar
The performance of deep learning models are driven by various parameters but to tune all of them every time, for every dataset, is a heuristic practice. In this paper, unlike the common practice of decaying the learning rate, we propose a step-wise training strategy where the learning rate and the batch size are tuned based on the dataset size. Here, the given dataset size is progressively increased during the training to boost the network performance without saturating the learning curve, after certain epochs. We conducted extensive experiments on multiple networks and datasets to validate the proposed training strategy. The experimental results proves our hypothesis that the learning rate, the batch size and the data size are interrelated and can improve the network accuracy if an optimal progressive stepwise training strategy is applied. The proposed strategy also the overall training computational cost is reduced.