Kyle Gillespie
Paper download is intended for registered attendees only, and is
subjected to the IEEE Copyright Policy. Any other use is strongly forbidden.
Papers from this author
Surface Material Dataset for Robotics Applications (SMDRA): A Dataset with Friction Coefficient and RGB-D for Surface Segmentation
Donghun Noh, Hyunwoo Nam, Min Sung Ahn, Hosik Chae, Sangjoon Lee, Kyle Gillespie, Dennis Hong
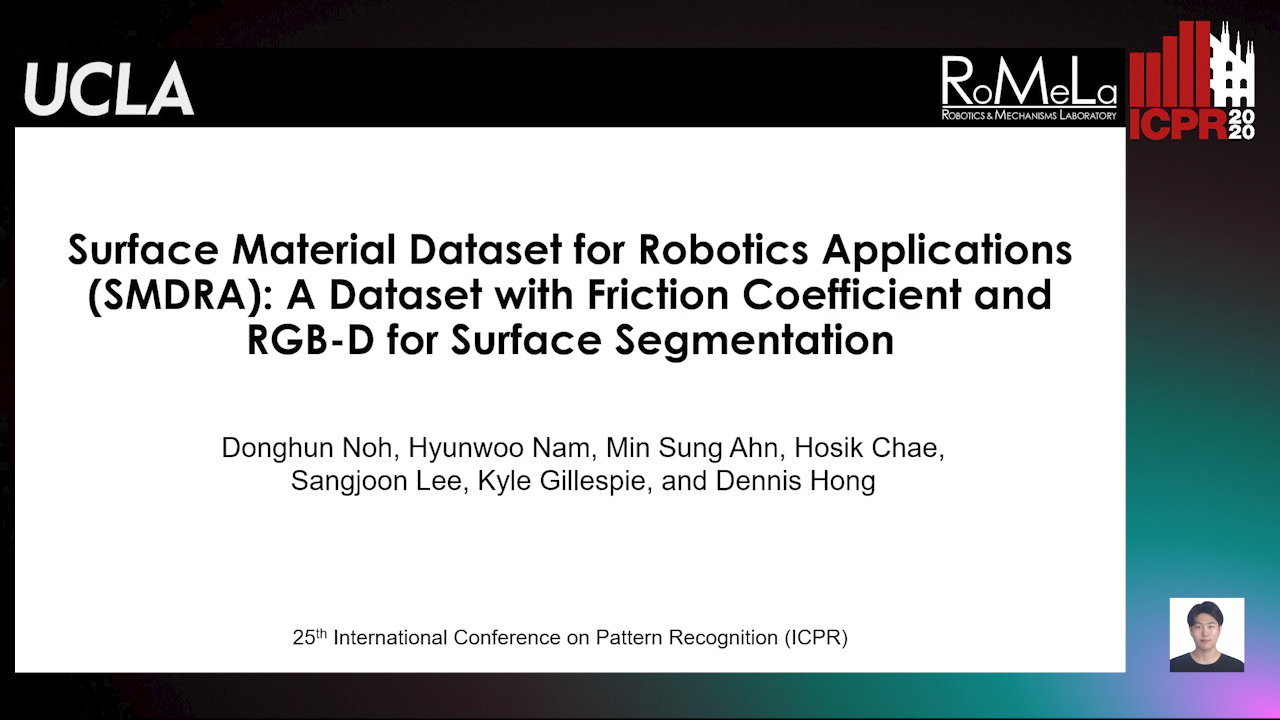
Auto-TLDR; A Surface Material Dataset for Robotics Applications
Abstract Slides Poster Similar
In this paper, we introduce the Surface Material Dataset for Robotics Applications (SMDRA), a collection of RGB color image, depth data, and pixel-wise friction coefficient data of 10 different materials for computer vision research specifically with robotics applications in mind that require physical contact between the robot and its environment such as robotic manipulators or walking robots. These selected surface materials are both easily accessible around our daily lives and cover a wide range of friction coefficients. Our dataset is unique in that while there is an abundance of RGB-D data due to the popularization of imaging sensors, additional pixel-wise aligned data of a different modality are not readily available. The depth data is collected by an active stereo camera which has shown promise on a variety of different robotic applications. In addition, this dataset is greatly expanded with friction coefficient data. Similarly to humans, this additional information can be helpful in ensuing proper decision making in tasks ranging from grasping orientation and strength to path determination in an unstructured environment. A newly developed friction measuring device was used to obtain this data. We verify that existing Convolutional Neural Network (CNN) architectures, the Fully Convolutional Network (FCN) and U-Net, can be trained on the SMDRA. This result demonstrates that the SMDRA can be utilized to train a neural network model for segmentation and these different modes are not just additional information, but valuable modes that researchers can incorporate and exploit when applying computer vision algorithms on robotic platforms.