Liuan Wang
Paper download is intended for registered attendees only, and is
subjected to the IEEE Copyright Policy. Any other use is strongly forbidden.
Papers from this author
NAS-EOD: An End-To-End Neural Architecture Search Method for Efficient Object Detection
Huigang Zhang, Liuan Wang, Jun Sun, Li Sun, Hiromichi Kobashi, Nobutaka Imamura
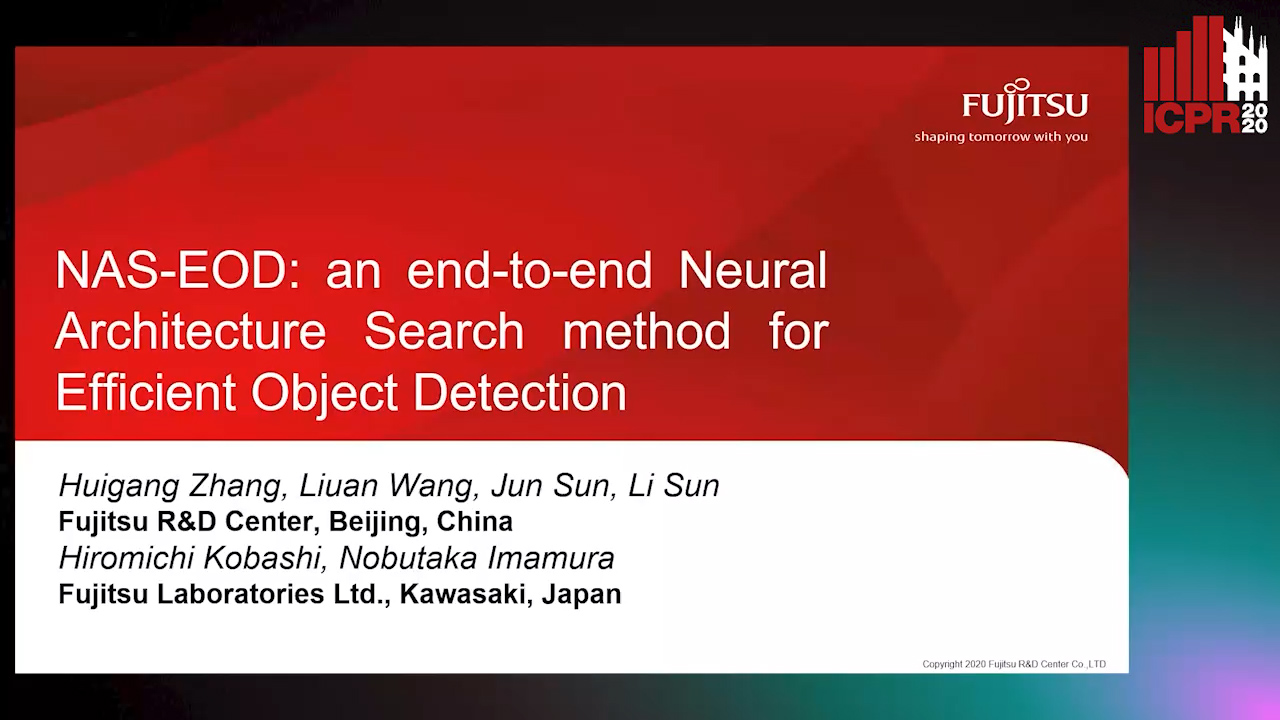
Auto-TLDR; NAS-EOD: Neural Architecture Search for Object Detection on Edge Devices
Model efficiency for object detection has become more and more important recently, especially when intelligent mobile devices are more and more convenient and developed today. Current small models for this task is either extended from the models for classification task, or pruned directly on the basis of large models. These pipelines are not task-specific or data-oriented so that their performance are not good enough for users. In this work, we propose a neural architecture search (NAS) method to build a detection model automatically that can perform well on edge devices. Specifically, the proposed method supports the search of not only multi-scale feature network, but also backbone network. This enables us to search out a global optimal model. To the best of our knowledge, it is a first attempt for searching an overall detection model via NAS. Additionally, we add latency information into the main objective during performance estimation, so that the search process can find a final model suitable for edge devices. Experiments on the PASCAL VOC benchmark indicate that the searched model (named NAS-EOD) can get good accuracy even without ImageNet pre-training. When using ImageNet pre-training, our model is superior to state-of-the-art small object detection models.