Haitao Yu
Paper download is intended for registered attendees only, and is
subjected to the IEEE Copyright Policy. Any other use is strongly forbidden.
Papers from this author
Edge-Aware Monocular Dense Depth Estimation with Morphology
Zhi Li, Xiaoyang Zhu, Haitao Yu, Qi Zhang, Yongshi Jiang
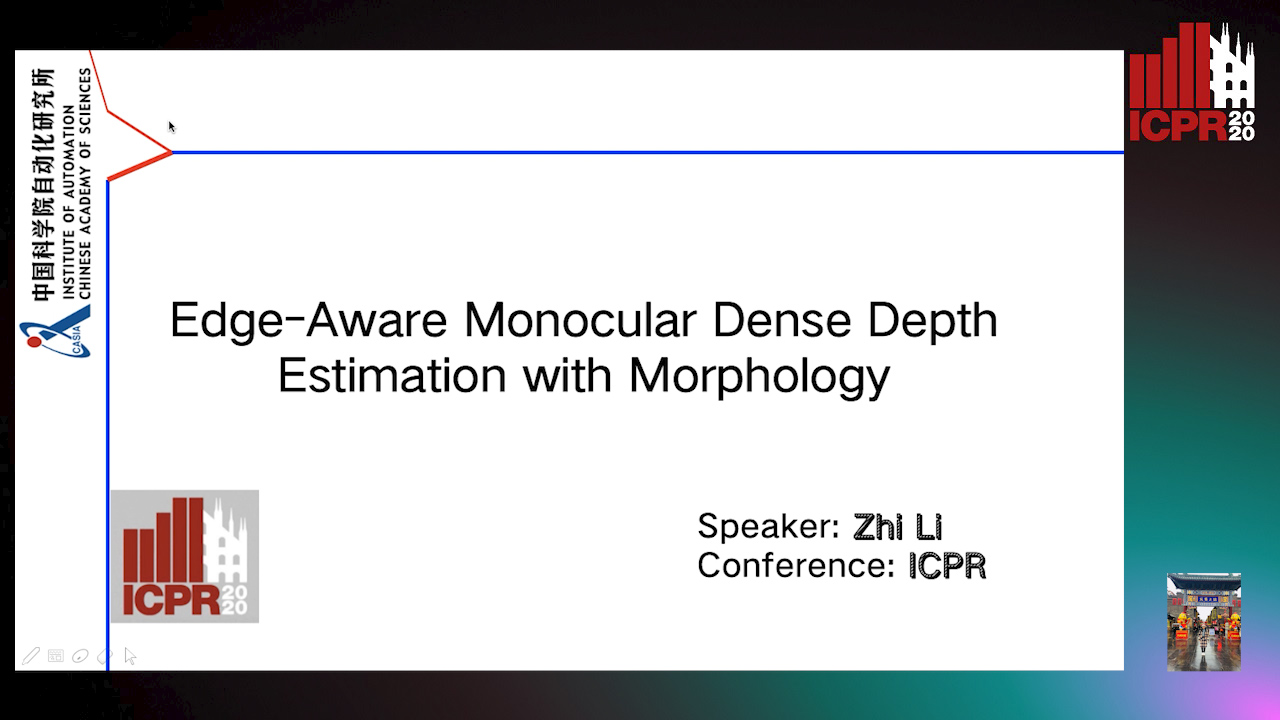
Auto-TLDR; Spatio-Temporally Smooth Dense Depth Maps Using Only a CPU
Abstract Slides Poster Similar
Dense depth maps play an important role in Computer Vision and AR (Augmented Reality). For CV applications, a dense depth map is the cornerstone of 3D reconstruction allowing real objects to be precisely displayed in the computer. And Dense depth maps can handle correct occlusion relationships between virtual content and real objects for better user experience in AR. However, the complicated computation limits the development of computing dense depth maps. We present a novel algorithm that produces low latency, spatio-temporally smooth dense depth maps using only a CPU. The depth maps exhibit sharp discontinuities at depth edges in low computational complexity ways. Our algorithm obtains the sparse SLAM reconstruction first, then extracts coarse depth edges from a down-sampled RGB image by morphology operations. Next, we thin the depth edges and align them with image edges. Finally, a Warm-Start initialization scheme and an improved optimization solver are adopted to accelerate convergence. We evaluate our proposal quantitatively and the result shows improvements on the accuracy of depth map with respect to other state-of-the-art and baseline techniques.