Ying Gu
Paper download is intended for registered attendees only, and is
subjected to the IEEE Copyright Policy. Any other use is strongly forbidden.
Papers from this author
Detecting Objects with High Object Region Percentage
Fen Fang, Qianli Xu, Liyuan Li, Ying Gu, Joo-Hwee Lim
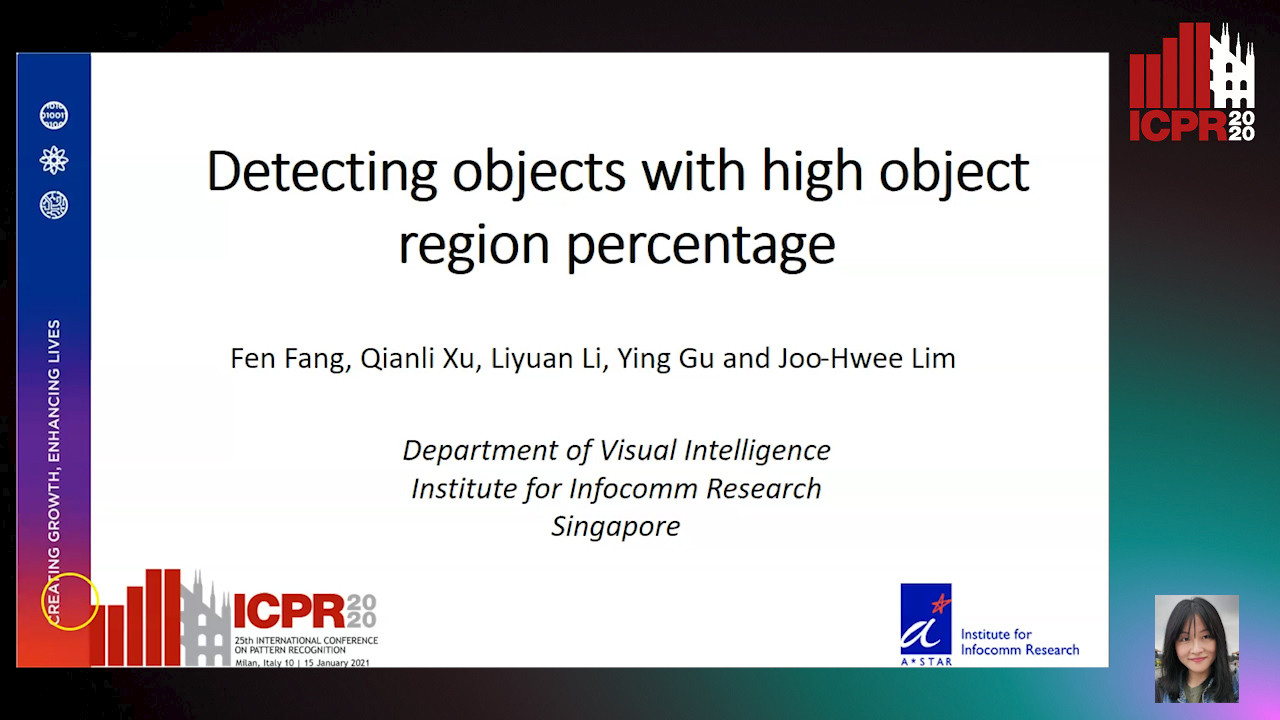
Auto-TLDR; Faster R-CNN for High-ORP Object Detection
Abstract Slides Poster Similar
Object shape is a subtle but important factor for object detection. It has been observed that the object-region-percentage (ORP) can be utilized to improve detection accuracy for elongated objects, which have much lower ORPs than other types of objects. In this paper, we propose an approach to improve the detection performance for objects whose ORPs are relatively higher.To address the problem of high-ORP object detection, we propose a method consisting of three steps. First, we adjust the ground truth bounding boxes of high-ORP objects to an optimal range. Second, we train an object detector, Faster R-CNN, based on adjusted bounding boxes to achieve high recall. Finally, we train a DCNN to learn the adjustment ratios towards four directions and adjust detected bounding boxes of objects to get better localization for higher precision. We evaluate the effectiveness of our method on 12 high-ORP objects in COCO and 8 objects in a proprietary gearbox dataset. The experimental results show that our method can achieve state-of-the-art performance on these objects while costing less resources in training and inference stages.