Wenxin Ma
Paper download is intended for registered attendees only, and is
subjected to the IEEE Copyright Policy. Any other use is strongly forbidden.
Papers from this author
PointDrop: Improving Object Detection from Sparse Point Clouds Via Adversarial Data Augmentation
Wenxin Ma, Jian Chen, Qing Du, Wei Jia
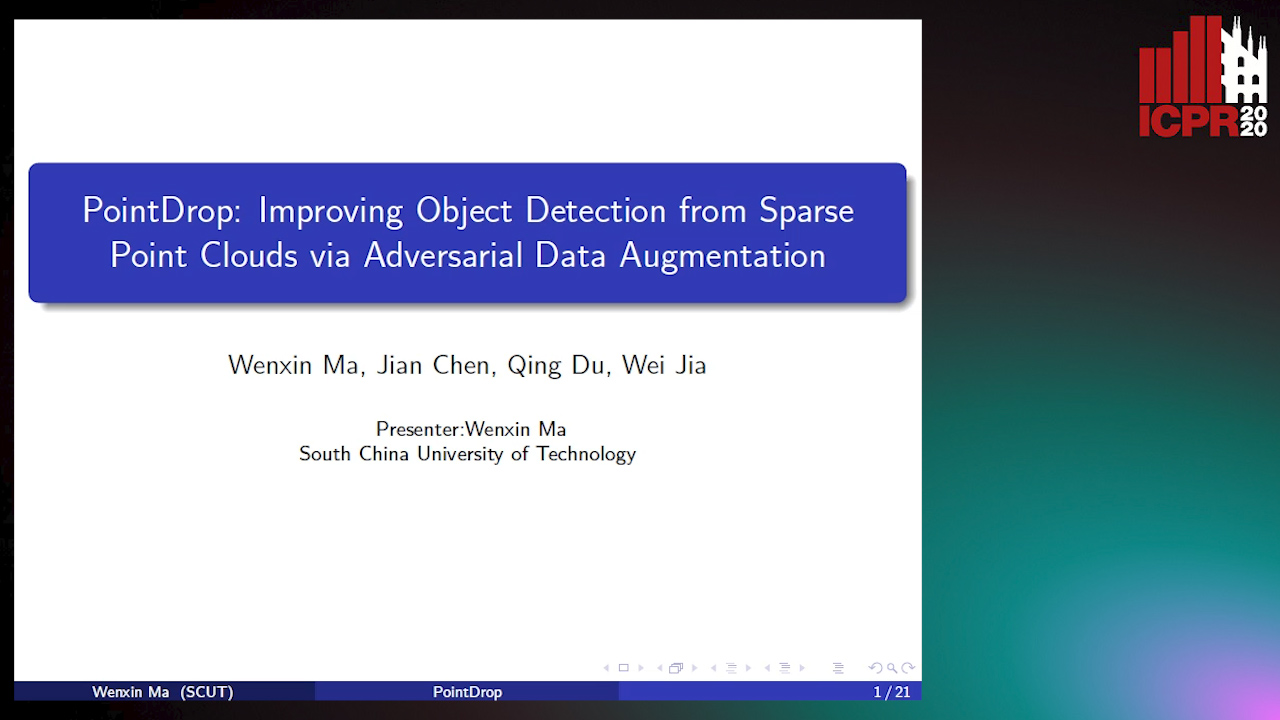
Auto-TLDR; PointDrop: Improving Robust 3D Object Detection to Sparse Point Clouds
Abstract Slides Poster Similar
Current 3D object detection methods achieve accurate and efficient results on the standard point cloud dataset. However, in real-world applications, due to the expensive cost of obtaining the annotated 3D object detection data, we expect to directly apply the model trained on the standard dataset to real-world scenarios. This strategy may fail because the point cloud samples obtained in the real-world scenarios may be much sparser due to various reasons (occlusion, low reflectivity of objects and fewer laser beams) and existing methods do not consider the limitations of their models on sparse point clouds. To improve the robustness of an object detector to sparser point clouds, we propose PointDrop, which learns to drop the features of some key points in the point clouds to generate challenging sparse samples for data augmentation. Moreover, PointDrop is able to adjust the difficulty of the generated samples based on the capacity of the detector and thus progressively improve the performance of the detector. We create two sparse point clouds datasets from the KITTI dataset to evaluate our method, and the experimental results show that PointDrop significantly improves the robustness of the detector to sparse point clouds.