Zihan Wang
Paper download is intended for registered attendees only, and is
subjected to the IEEE Copyright Policy. Any other use is strongly forbidden.
Papers from this author
SIDGAN: Single Image Dehazing without Paired Supervision
Pan Wei, Xin Wang, Lei Wang, Ji Xiang, Zihan Wang
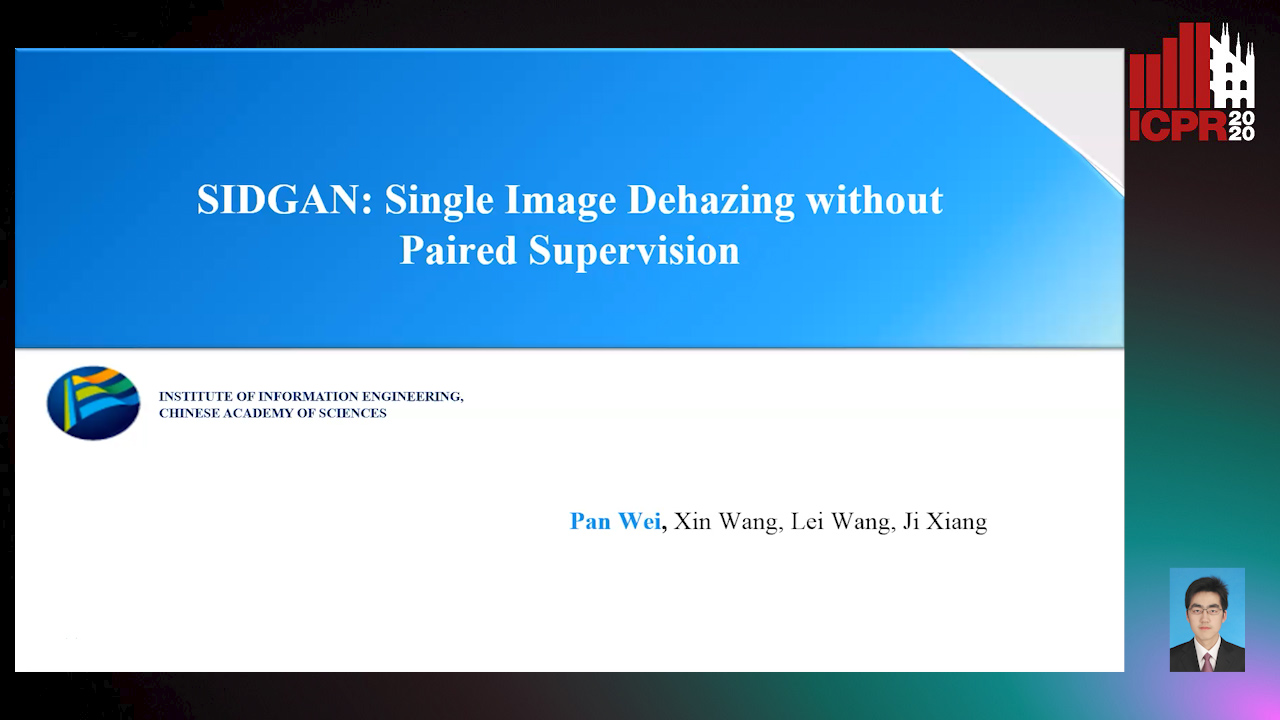
Auto-TLDR; DehazeGAN: An End-to-End Generative Adversarial Network for Image Dehazing
Abstract Slides Poster Similar
Single image dehazing is challenging without scene airlight and transmission map. Most of existing dehazing algorithms tend to estimate key parameters based on manual designed priors or statistics, which may be invalid in some scenarios. Although deep learning-based dehazing methods provide an effective solution, most of them rely on paired training datasets, which are prohibitively difficult to be collected in real world. In this paper, we propose an effective end-to-end generative adversarial network for image dehazing, named DehazeGAN. The proposed DehazeGAN adopts a U-net architecture with a novel color-consistency loss derived from dark channel prior and perceptual loss, which can be trained in an unsupervised fashion without paired synthetic datasets. We create a RealHaze dataset for network training, including 4,000 outdoor hazy images and 4,000 haze-free images. Extensive experiments demonstrate that our proposed DehazeGAN achieves better performance than existing state-of-the-art methods on both synthetic datasets and real-world datasets in terms of PSNR, SSIM, and subjective visual experience.