David-Alexandre Beaupre
Paper download is intended for registered attendees only, and is
subjected to the IEEE Copyright Policy. Any other use is strongly forbidden.
Papers from this author
Domain Siamese CNNs for Sparse Multispectral Disparity Estimation
David-Alexandre Beaupre, Guillaume-Alexandre Bilodeau
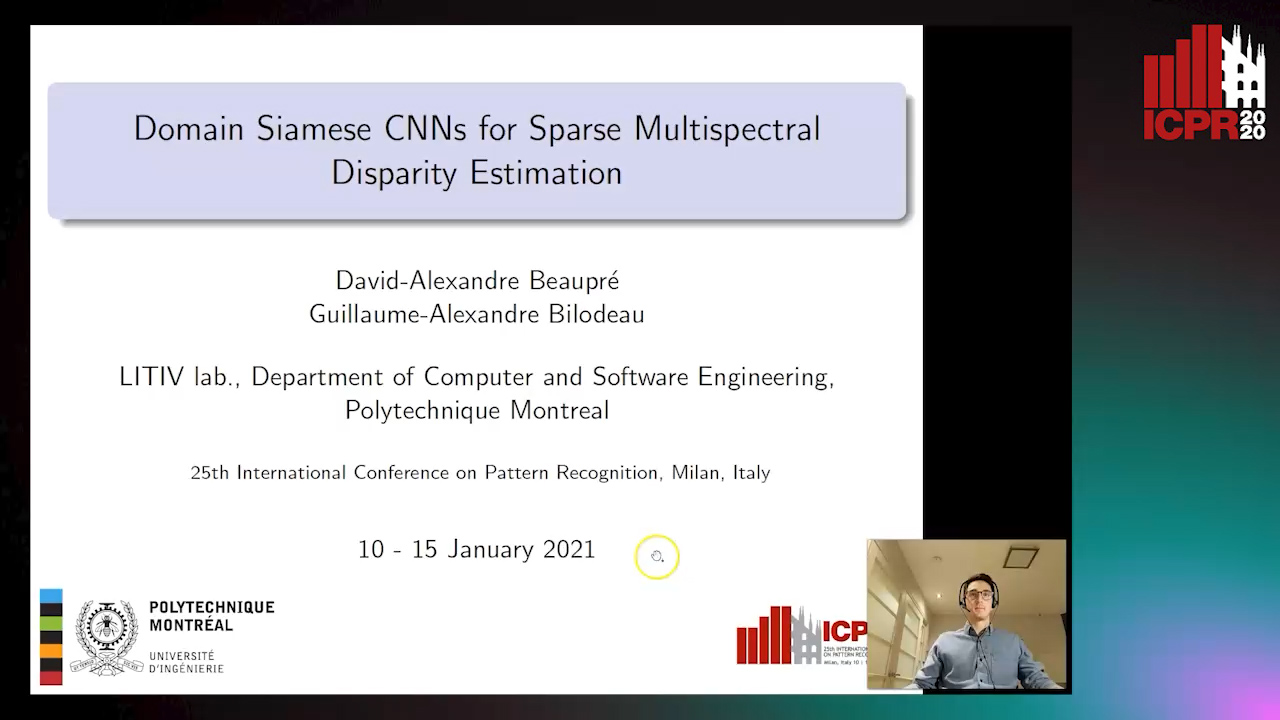
Auto-TLDR; Multispectral Disparity Estimation between Thermal and Visible Images using Deep Neural Networks
Abstract Slides Poster Similar
Multispectral disparity estimation is a difficult task for many reasons: it as all the same challenges as traditional visible-visible disparity estimation (occlusions, repetitive patterns, textureless surfaces), in addition of having very few common visual information between images (e.g. color information vs. thermal information). In this paper, we propose a new CNN architecture able to do disparity estimation between images from different spectrum, namely thermal and visible in our case. Our proposed model takes two patches as input and proceeds to do domain feature extraction for each of them. Features from both domains are then merged with two fusion operations, namely correlation and concatenation. These merged vectors are then forwarded to their respective classification heads, which are responsible for classifying the inputs as being same or not. Using two merging operations gives more robustness to our feature extraction process, which leads to more precise disparity estimation. Our method was tested using the publicly available LITIV 2014 and LITIV 2018 datasets, and showed best results when compared to other state of the art methods.