Luigi Di Stefano
Paper download is intended for registered attendees only, and is
subjected to the IEEE Copyright Policy. Any other use is strongly forbidden.
Papers from this author
Effective Deployment of CNNs for 3DoF Pose Estimation and Grasping in Industrial Settings
Daniele De Gregorio, Riccardo Zanella, Gianluca Palli, Luigi Di Stefano
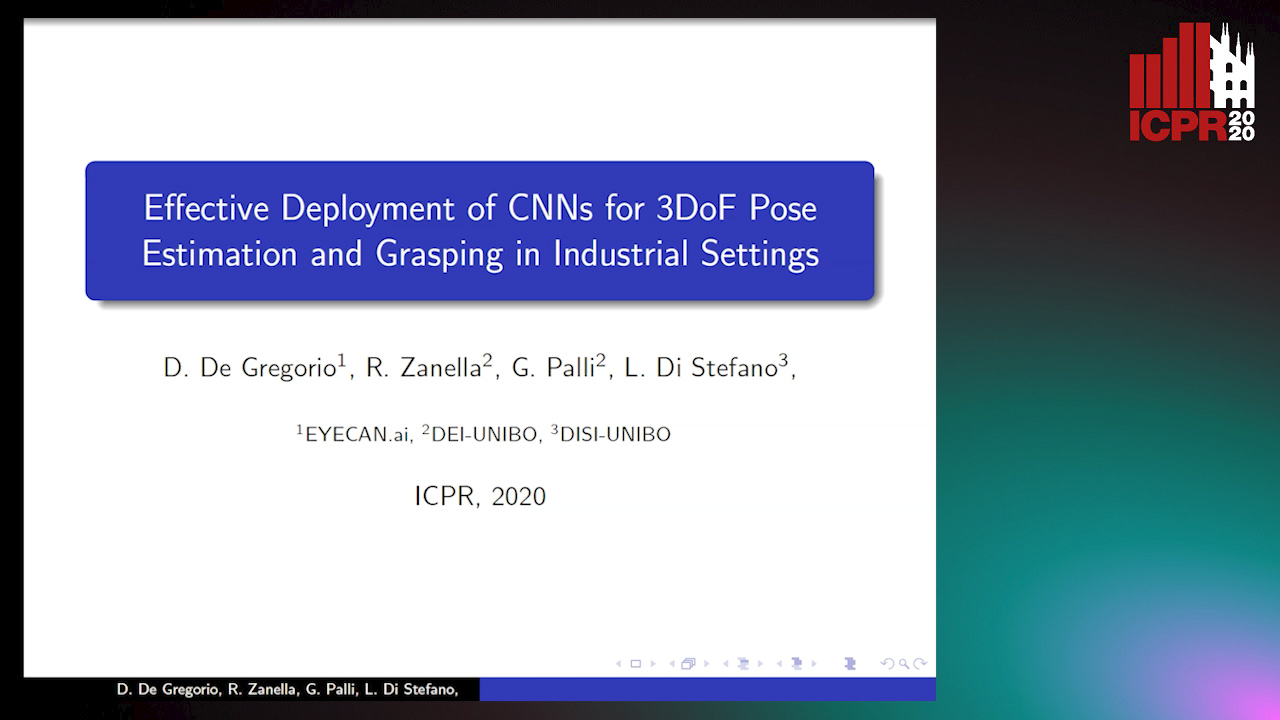
Auto-TLDR; Automated Deep Learning for Robotic Grasping Applications
Abstract Slides Poster Similar
In this paper we investigate how to effectively deploy deep learning in practical industrial settings, such as robotic grasping applications. When a deep-learning based solution is proposed, usually lacks of any simple method to generate the training data. In the industrial field, where automation is the main goal, not bridging this gap is one of the main reasons why deep learning is not as widespread as it is in the academic world. For this reason, in this work we developed a system composed by a 3-DoF Pose Estimator based on Convolutional Neural Networks (CNNs) and an effective procedure to gather massive amounts of training images in the field with minimal human intervention. By automating the labeling stage, we also obtain very robust systems suitable for production-level usage. An open source implementation of our solution is provided, alongside with the dataset used for the experimental evaluation.