Kai Andreas Metzger
Paper download is intended for registered attendees only, and is
subjected to the IEEE Copyright Policy. Any other use is strongly forbidden.
Papers from this author
A Fine-Grained Dataset and Its Efficient Semantic Segmentation for Unstructured Driving Scenarios
Kai Andreas Metzger, Peter Mortimer, Hans J "Joe" Wuensche
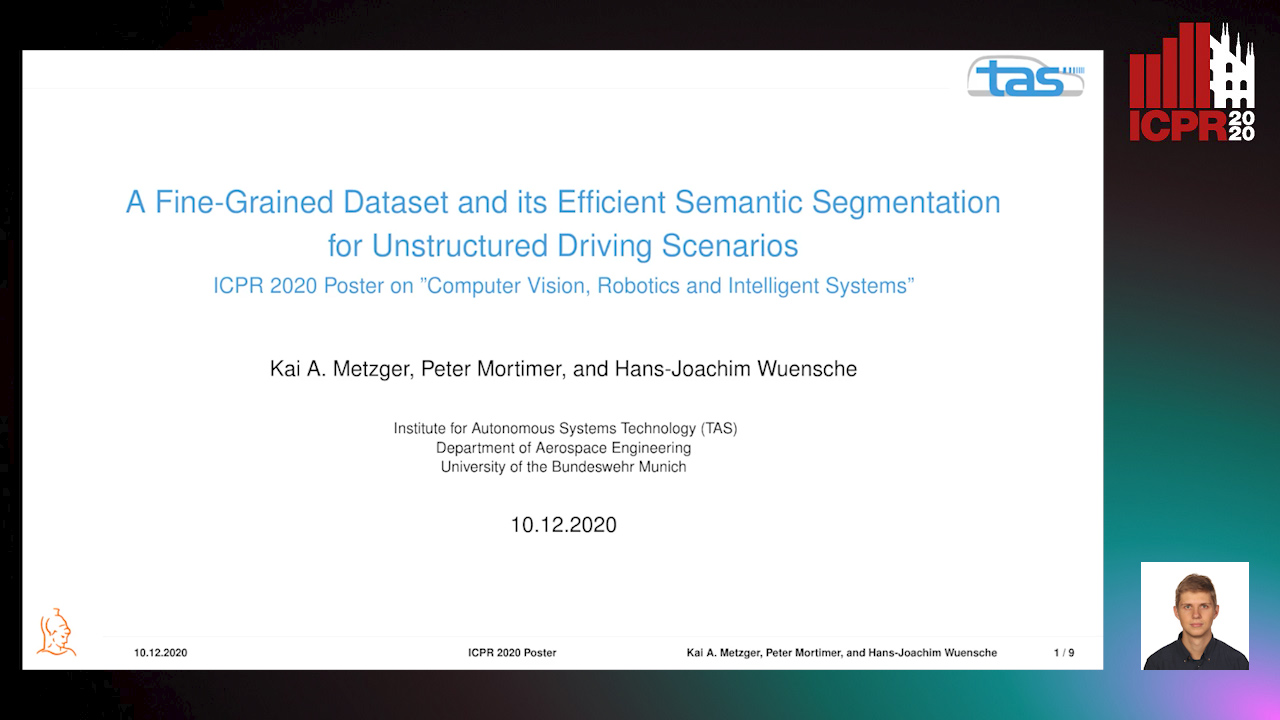
Auto-TLDR; TAS500: A Semantic Segmentation Dataset for Autonomous Driving in Unstructured Environments
Abstract Slides Poster Similar
Research in autonomous driving for unstructured environments suffers from a lack of semantically labeled datasets compared to its urban counterpart. Urban and unstructured outdoor environments are challenging due to the varying lighting and weather conditions during a day and across seasons. In this paper, we introduce TAS500, a novel semantic segmentation dataset for autonomous driving in unstructured environments. TAS500 offers fine-grained vegetation and terrain classes to learn drivable surfaces and natural obstacles in outdoor scenes effectively. We evaluate the performance of modern semantic segmentation models with an additional focus on their efficiency. Our experiments demonstrate the advantages of fine-grained semantic classes to improve the overall prediction accuracy, especially along the class boundaries. The dataset, code, and pretrained model are available online.