Qi Cheng
Paper download is intended for registered attendees only, and is
subjected to the IEEE Copyright Policy. Any other use is strongly forbidden.
Papers from this author
MagnifierNet: Learning Efficient Small-Scale Pedestrian Detector towards Multiple Dense Regions
Qi Cheng, Mingqin Chen, Yingjie Wu, Fei Chen, Shiping Lin
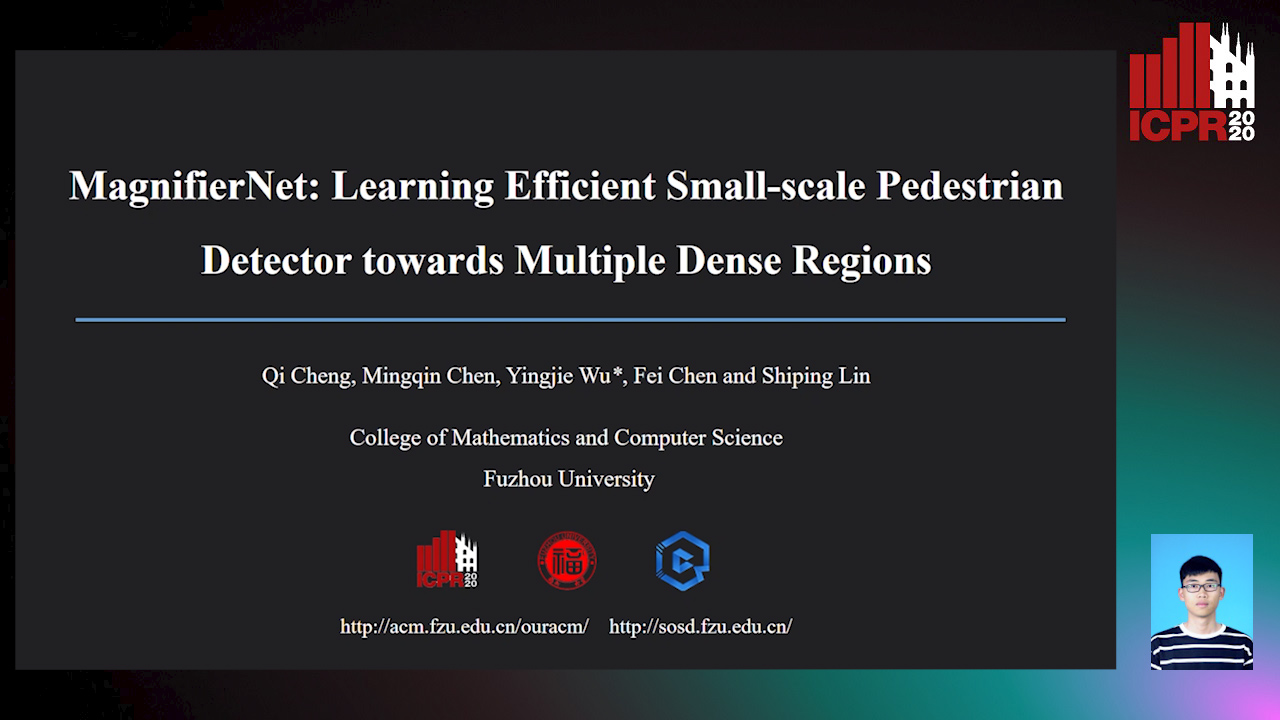
Auto-TLDR; MagnifierNet: A Simple but Effective Small-Scale Pedestrian Detection Towards Multiple Dense Regions
Abstract Slides Poster Similar
Despite the success of pedestrian detection, there is still a significant gap in the performance of the detection of pedestrians at different scales. Detecting small-scale pedestrians is extremely challenging due to the low resolution of their convolution features which is essential for downstream classifiers. To address this issue, we observed pedestrian datasets and found that pedestrians often gather together in crowded public places. Then we propose MagnifierNet, a simple but effective small-scale pedestrian detector towards multiple dense regions. MagnifierNet uses our proposed sweep-line based grouping algorithm to find dense regions based on the number of pedestrians in the grouped region. And we adopt a new definition of small-scale pedestrians through grid search and KL-divergence. Besides, our grouping method can also be used as a new strategy for pedestrian data augmentation. The ablation study demonstrates that MagnifierNet improves the representation of small-scale pedestrians. We validate the effectiveness of MagnifierNet on CityPersons and KITTI datasets. Experimental results show that MagnifierNet achieves the best small-scale pedestrian detection performance on CityPersons benchmark without any external data, and also achieves competitive performance for detecting small-scale pedestrians on KITTI dataset without bells and whistles.