Yihao Wang
Paper download is intended for registered attendees only, and is
subjected to the IEEE Copyright Policy. Any other use is strongly forbidden.
Papers from this author
Precise Temporal Action Localization with Quantified Temporal Structure of Actions
Chongkai Lu, Ruimin Li, Hong Fu, Bin Fu, Yihao Wang, Wai Lun Lo, Zheru Chi
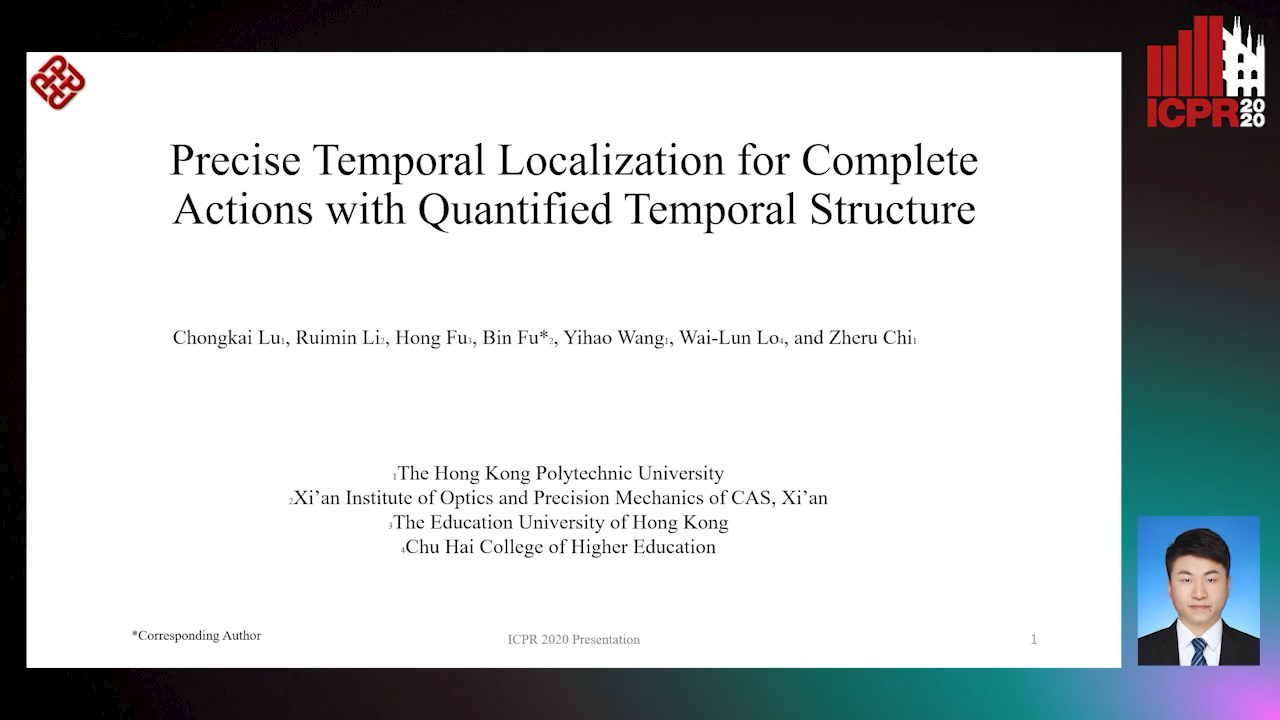
Auto-TLDR; Action progression networks for temporal action detection
Abstract Slides Poster Similar
Existing temporal action detection algorithms cannot distinguish complete and incomplete actions while this property is essential in many applications. To tackle this challenge, we proposed the action progression networks (APN), a novel model that predicts action progression of video frames with continuous numbers. Using the progression sequence of test video, on the top of the APN, a complete action searching algorithm (CAS) was designed to detect complete actions only. With the usage of frame-level fine-grained temporal structure modeling and detecting actions according to their whole temporal context, our framework can locate actions precisely and is good at avoiding incomplete action detection. We evaluated our framework on a new dataset (DFMAD-70) collected by ourselves which contains both complete and incomplete actions. Our framework got good temporal localization results with 95.77% average precision when the IoU threshold is 0.5. On the benchmark THUMOS14, an incomplete-ignostic dataset, our framework still obtain competitive performance. The code is available online at https://github.com/MakeCent/Action-Progression-Network