Jihun Park
Paper download is intended for registered attendees only, and is
subjected to the IEEE Copyright Policy. Any other use is strongly forbidden.
Papers from this author
Transitional Asymmetric Non-Local Neural Networks for Real-World Dirt Road Segmentation
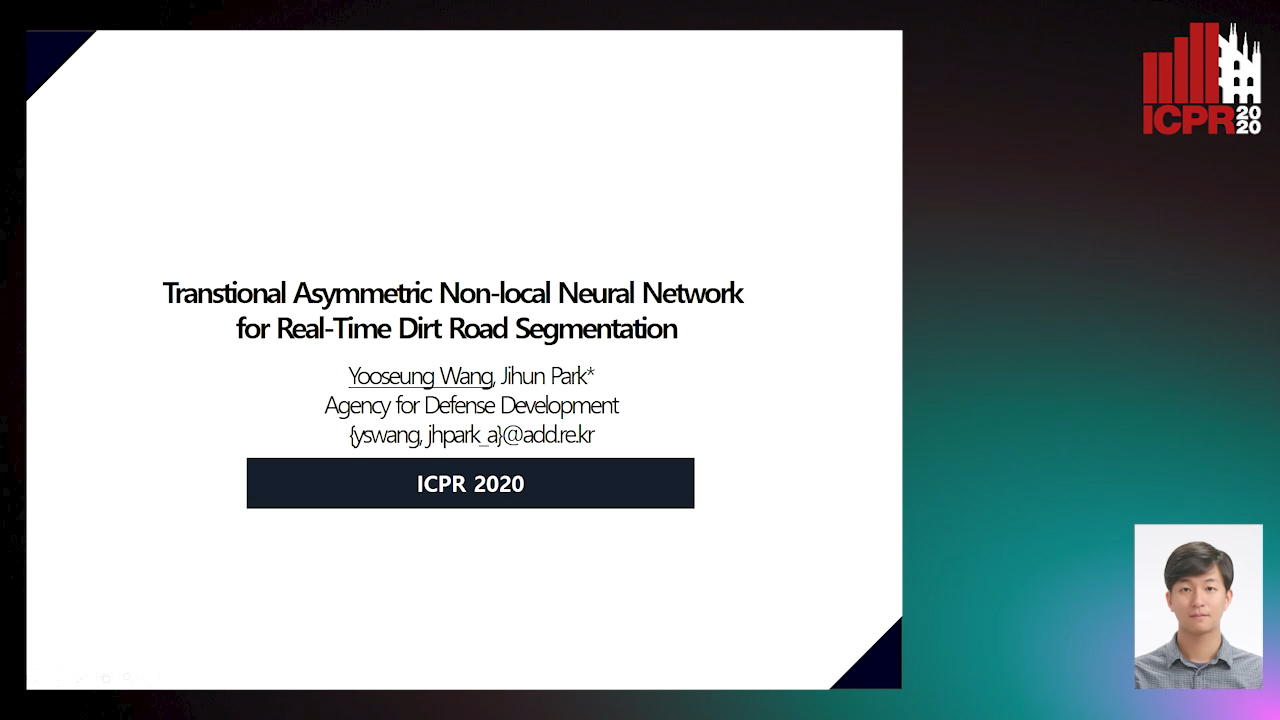
Auto-TLDR; Transitional Asymmetric Non-Local Neural Networks for Semantic Segmentation on Dirt Roads
Abstract Slides Poster Similar
Understanding images by predicting pixel-level semantic classes is a fundamental task in computer vision and is one of the most important techniques for autonomous driving. Recent approaches based on deep convolutional neural networks have dramatically improved the speed and accuracy of semantic segmentation on paved road datasets, however, dirt roads have yet to be systematically studied. Dirt roads do not contain clear boundaries between drivable and non-drivable regions; and thus, this difficulty must be overcome for the realization of fully autonomous vehicles. The key idea of our approach is to apply lightweight non-local blocks to reinforce stage-wise long-range dependencies in encoder-decoder style backbone networks. Experiments on 4,687 images of a dirt road dataset show that our transitional asymmetric non-local neural networks present a higher accuracy with lower computational costs compared to state-of-the-art models.