Lukas Frank
Paper download is intended for registered attendees only, and is
subjected to the IEEE Copyright Policy. Any other use is strongly forbidden.
Papers from this author
Multi-Task Learning for Calorie Prediction on a Novel Large-Scale Recipe Dataset Enriched with Nutritional Information
Robin Ruede, Verena Heusser, Lukas Frank, Monica Haurilet, Alina Roitberg, Rainer Stiefelhagen
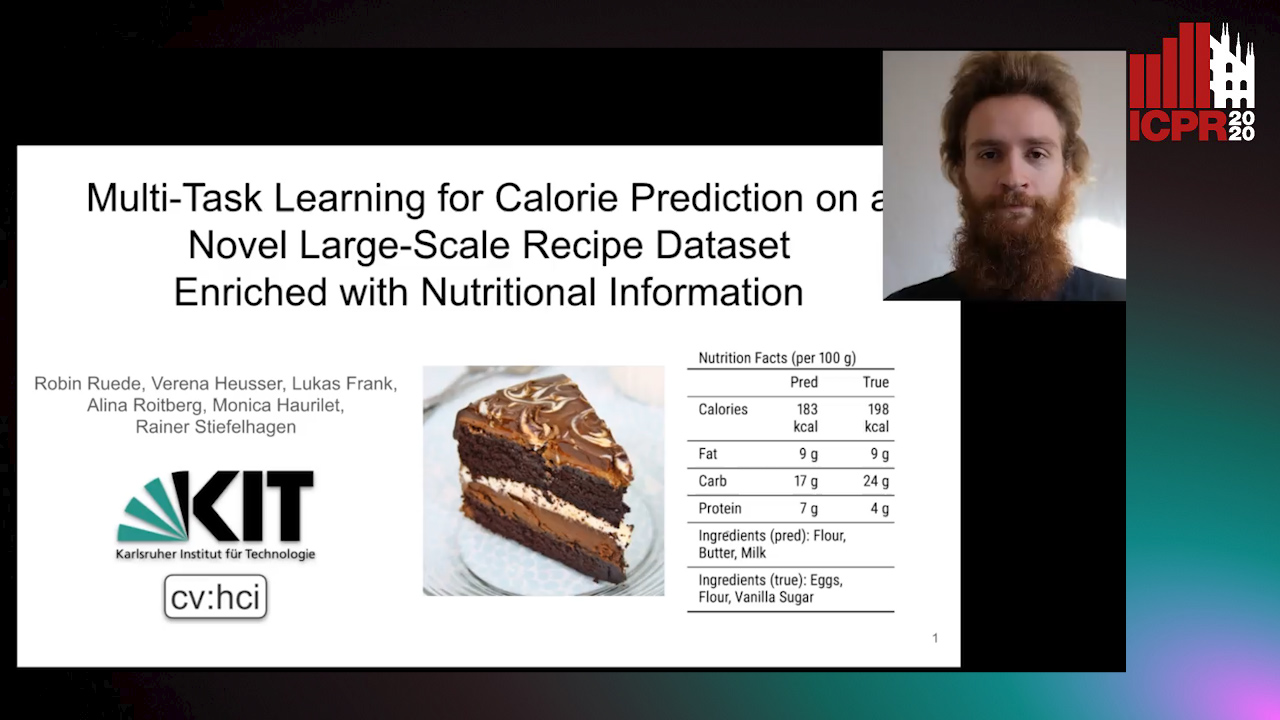
Auto-TLDR; Pic2kcal: Learning Food Recipes from Images for Calorie Estimation
Abstract Slides Poster Similar
A rapidly growing amount of content posted online, such as food recipes, opens doors to new exciting applications at the intersection of vision and language. In this work, we aim to estimate the calorie amount of a meal directly from an image by learning from recipes people have published on the Internet, thus skipping time-consuming manual data annotation. Since there are few large-scale publicly available datasets captured in unconstrained environments, we propose the pic2kcal benchmark comprising 308,000 images from over 70,000 recipes including photographs, ingredients and instructions. To obtain nutritional information of the ingredients and automatically determine the ground-truth calorie value, we match the items in the recipes with structured information from a food item database. We evaluate various neural networks for regression of the calorie quantity and extend them with the multi-task paradigm. Our learning procedure combines the calorie estimation with prediction of proteins, carbohydrates, and fat amounts as well as a multi-label ingredient classification. Our experiments demonstrate clear benefits of multi-task learning for calorie estimation, surpassing the single-task calorie regression by 9.9%. To encourage further research on this task, we make the code for generating the dataset and the models publicly available.