Qi Wan
Paper download is intended for registered attendees only, and is
subjected to the IEEE Copyright Policy. Any other use is strongly forbidden.
Papers from this author
Feature Embedding Based Text Instance Grouping for Largely Spaced and Occluded Text Detection
Pan Gao, Qi Wan, Renwu Gao, Linlin Shen
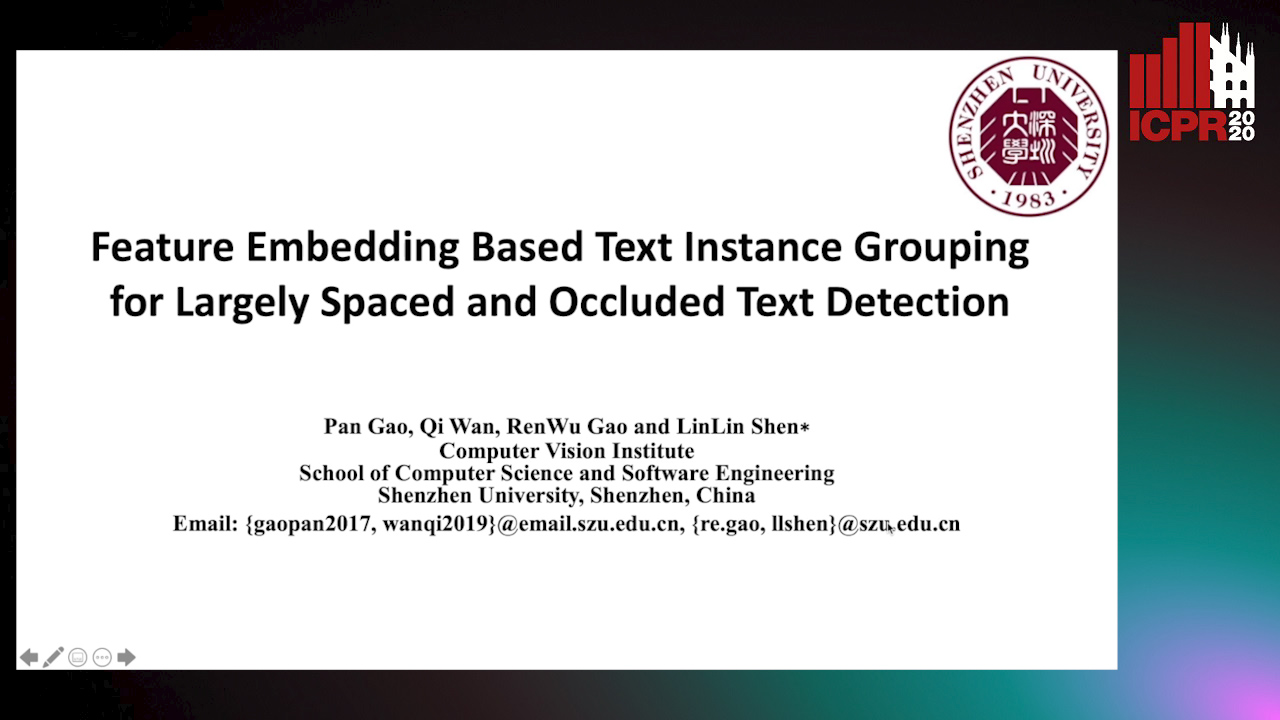
Auto-TLDR; Text Instance Embedding Based Feature Embeddings for Multiple Text Instance Grouping
Abstract Slides Poster Similar
A text instance can be easily detected as multiple ones due to the large space between texts/characters, curved shape and partial occlusion. In this paper, a feature embedding based text instance grouping algorithm is proposed to solve this problem. To learn the feature space, a TIEM (Text Instance Embedding Module) is trained to minimize the within instance scatter and maximize the between instance scatter. Similarity between different text instances are measured in the feature space and merged if they meet certain conditions. Experimental results show that our approach can effectively connect text regions that belong to the same text instance. Competitive performance of our approach has been achieved on CTW1500, Total-Text, IC15 and a subset consists of texts selected from the three datasets, with large spacing and occlusions.