Liu Peiyu
Paper download is intended for registered attendees only, and is
subjected to the IEEE Copyright Policy. Any other use is strongly forbidden.
Papers from this author
Feature-Aware Unsupervised Learning with Joint Variational Attention and Automatic Clustering
Wang Ru, Lin Li, Peipei Wang, Liu Peiyu
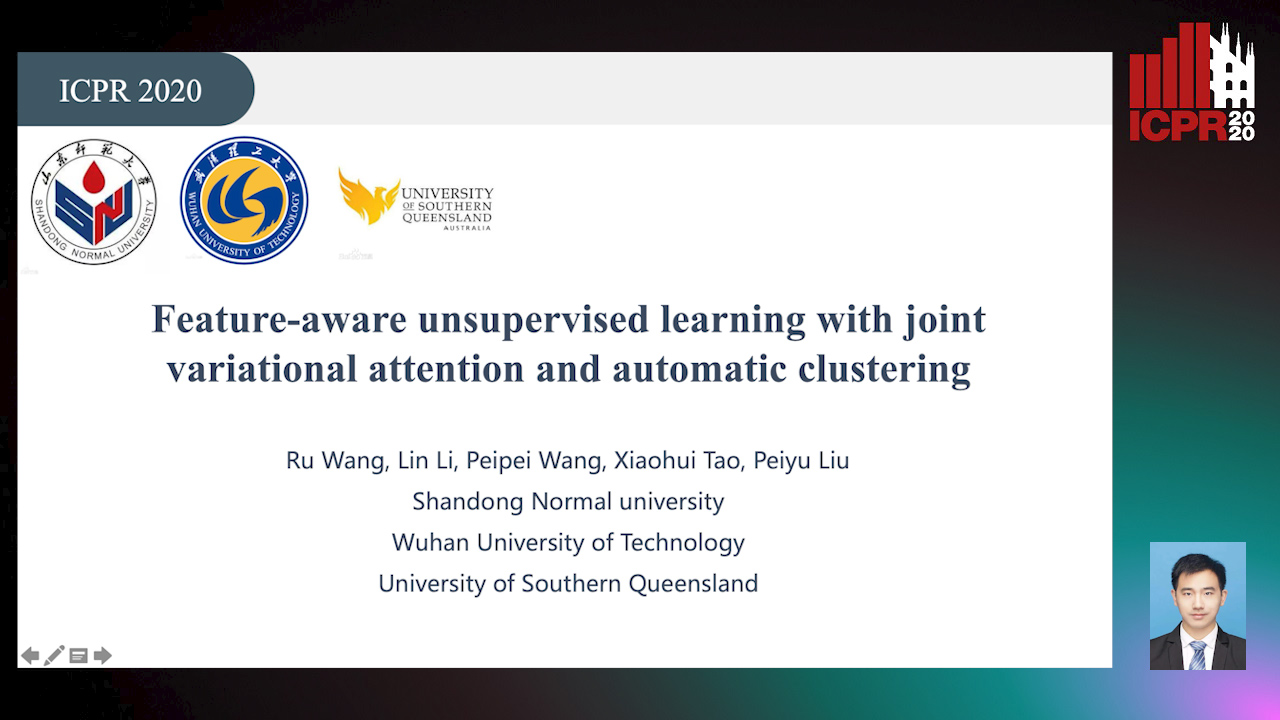
Auto-TLDR; Deep Variational Attention Encoder-Decoder for Clustering
Abstract Slides Poster Similar
Deep clustering aims to cluster unlabeled real-world samples by mining deep feature representation. Most of existing methods remain challenging when handling high-dimensional data and simultaneously exploring the complementarity of deep feature representation and clustering. In this paper, we propose a novel Deep Variational Attention Encoder-decoder for Clustering (DVAEC). Our DVAEC improves the representation learning ability by fusing variational attention. Specifically, we design a feature-aware automatic clustering module to mitigate the unreliability of similarity calculation and guide network learning. Besides, to further boost the performance of deep clustering from a global perspective, we define a joint optimization objective to promote feature representation learning and automatic clustering synergistically. Extensive experimental results show the promising performance achieved by our DVAEC on six datasets comparing with several popular baseline clustering methods.