Maria Tzelepi
Paper download is intended for registered attendees only, and is
subjected to the IEEE Copyright Policy. Any other use is strongly forbidden.
Papers from this author
Efficient Online Subclass Knowledge Distillation for Image Classification
Maria Tzelepi, Nikolaos Passalis, Anastasios Tefas
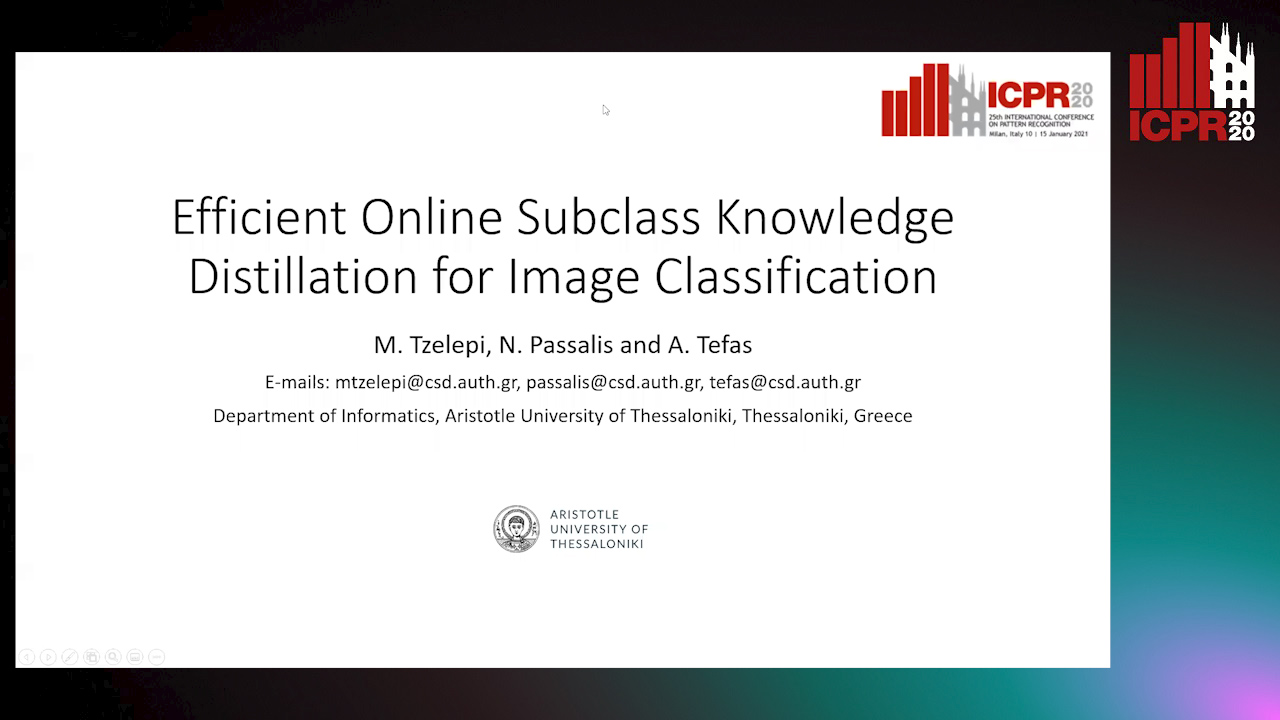
Auto-TLDR; OSKD: Online Subclass Knowledge Distillation
Abstract Slides Poster Similar
Deploying state-of-the-art deep learning models on embedded systems dictates certain storage and computation limitations. During the recent few years Knowledge Distillation (KD) has been recognized as a prominent approach to address this issue. That is, KD has been effectively proposed for training fast and compact deep learning models by transferring knowledge from more complex and powerful models. However, knowledge distillation, in its conventional form, involves multiple stages of training, rendering it a computationally and memory demanding procedure. In this paper, a novel single-stage self knowledge distillation method is proposed, namely Online Subclass Knowledge Distillation (OSKD), that aims at revealing the similarities inside classes, improving the performance of any deep neural model in an online manner. Hence, as opposed to existing online distillation methods, we are able to acquire further knowledge from the model itself, without building multiple identical models or using multiple models to teach each other, rendering the OSKD approach more efficient. The experimental evaluation on two datasets validates that the proposed method improves the classification performance.