Yafeng Zhou
Paper download is intended for registered attendees only, and is
subjected to the IEEE Copyright Policy. Any other use is strongly forbidden.
Papers from this author
Dual Loss for Manga Character Recognition with Imbalanced Training Data
Yonggang Li, Yafeng Zhou, Yongtao Wang, Xiaoran Qin, Zhi Tang
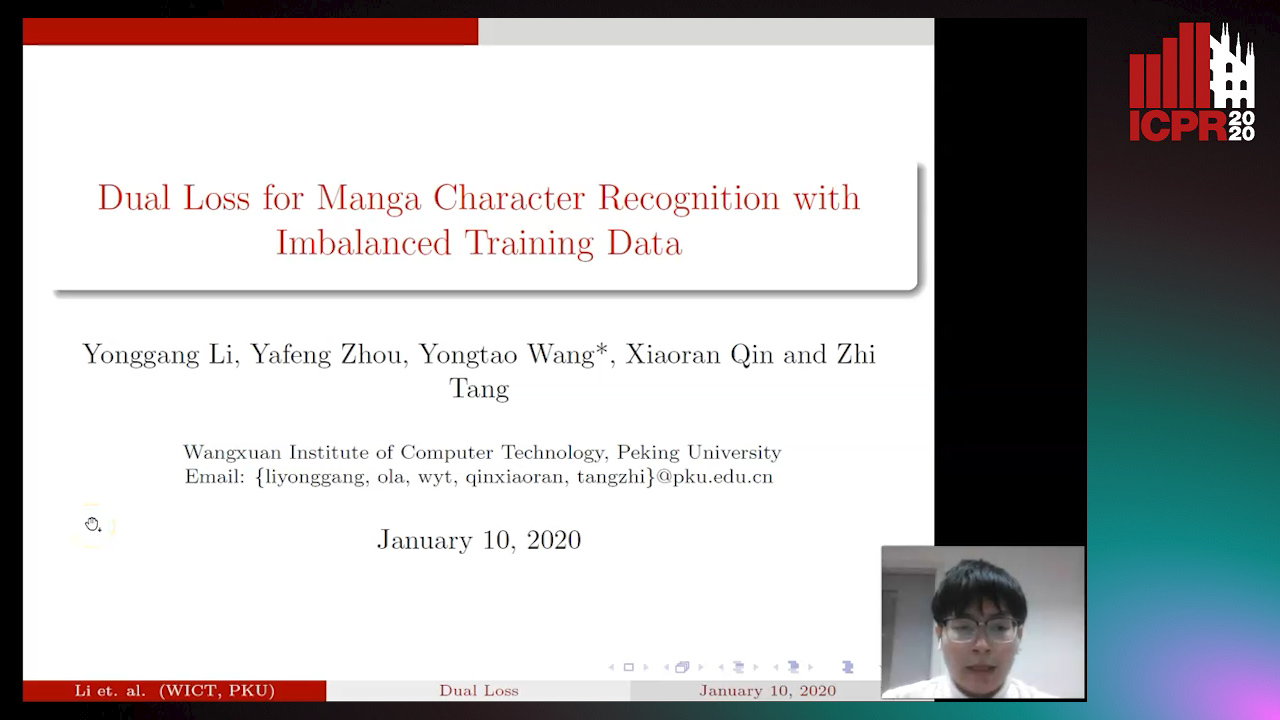
Auto-TLDR; Dual Adaptive Re-weighting Loss for Manga Character Recognition
Abstract Slides Poster Similar
Manga character recognition is a key technology for manga character retrieval and verfication. This task is very challenging since the manga character images have a long-tailed distribution and large quality variations. Training models with cross-entropy softmax loss on such imbalanced data would introduce biases to feature and class weight norm. To handle this problem, we propose a novel dual loss which is the sum of two losses: dual ring loss and dual adaptive re-weighting loss. Dual ring loss combines weight and feature soft normalization and serves as a regularization term to softmax loss. Dual adaptive re-weighting loss re-weights softmax loss according to the norm of both feature and class weight. With the proposed losses, we have achieved encouraging results on Manga109 benchmark. Specifically, compared with the baseline softmax loss, our method improves the character retrieval mAP from 35.72% to 38.88% and the character verification accuracy from 87.00% to 88.50%.