Rao Muhammad Umer
Paper download is intended for registered attendees only, and is
subjected to the IEEE Copyright Policy. Any other use is strongly forbidden.
Papers from this author
Deep Iterative Residual Convolutional Network for Single Image Super-Resolution
Rao Muhammad Umer, Gian Luca Foresti, Christian Micheloni
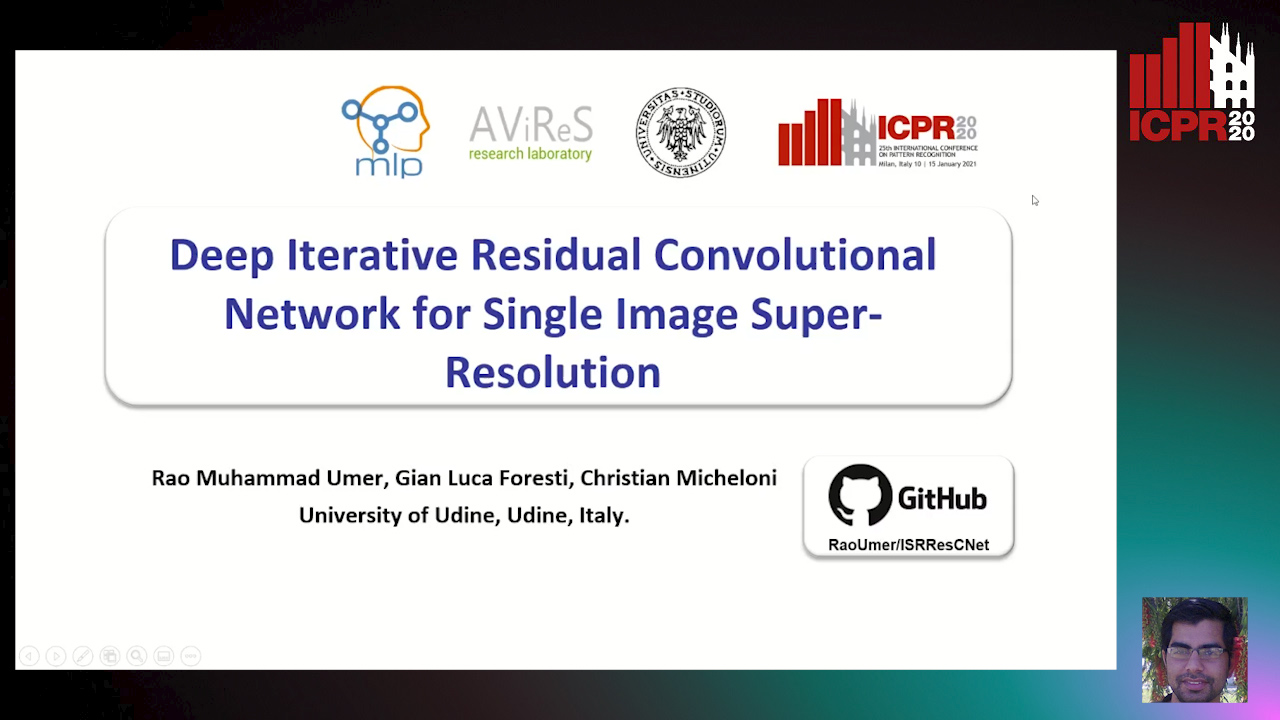
Auto-TLDR; ISRResCNet: Deep Iterative Super-Resolution Residual Convolutional Network for Single Image Super-resolution
Deep convolutional neural networks (CNNs) have recently achieved great success for single image super-resolution (SISR) task due to their powerful feature representation capabilities. Most recent deep learning based SISR methods focus on designing deeper / wider models to learn the non-linear mapping between low-resolution (LR) inputs and the high-resolution (HR) outputs. These existing SR methods do not take into account the image observation (physical) model and thus require a large number of network's trainable parameters with a huge volume of training data. To address these issues, we propose a deep Iterative Super-Resolution Residual Convolutional Network (ISRResCNet) that exploits the powerful image regularization and large-scale optimization techniques by training the deep network in an iterative manner with a residual learning approach. Extensive experimental results on various super-resolution benchmarks demonstrate that our method with a few trainable parameters improves results for different scaling factors in comparison with the state-of-art methods.