Linlin Tang
Paper download is intended for registered attendees only, and is
subjected to the IEEE Copyright Policy. Any other use is strongly forbidden.
Papers from this author
A Riemannian Framework for Detecting Stimulus-Relevant Fiber Pathways
Jingyong Su, Linlin Tang, Zhipeng Yang, Mengmeng Guo
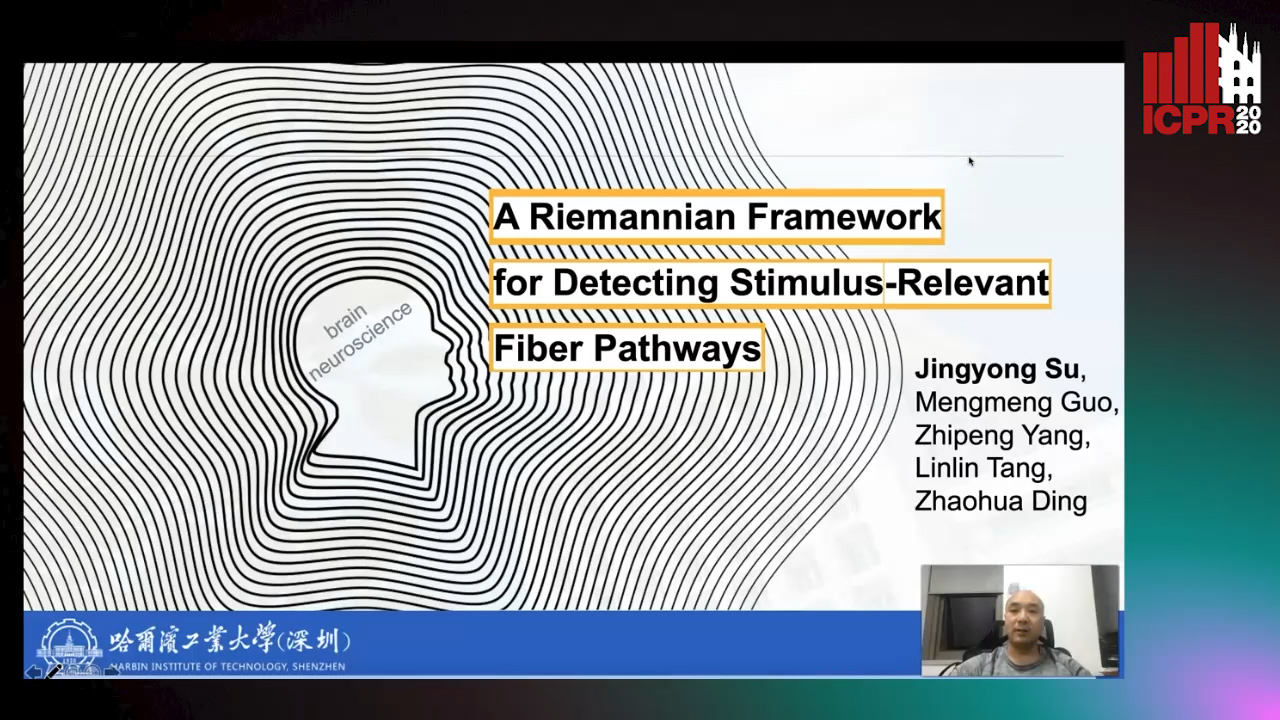
Auto-TLDR; Clustering Task-Specific Fiber Pathways in Functional MRI using BOLD Signals
Functional MRI based on blood oxygenation level-dependent (BOLD) contrast is well established as a neuro-imaging technique for detecting neural activity in the cortex of the human brain. Recent studies have shown that variations of BOLD signals in white matter are also related to neural activities both in resting state and under functional loading. We develop a comprehensive framework of detecting task-specific fiber pathways. We not only study fiber tracts as open curves with different physical features (shape, scale, orientation and position), but also incorporate the BOLD signals transmitted along them to find stimulus-relevant pathways. Specifically, we propose a novel Riemannian metric, which is a weighted sum of distances in product space of shapes and functions. This metric provides both a cost function for registration and a proper distance for comparison. Experimental results on real data have shown that we can cluster fiber pathways correctly by evaluating correlations between BOLD signals and stimuli, temporal variations and power spectra of them. The proposed framework can also be easily generalized to various applications where multi-modality data exist.