Stephen Bonner
Paper download is intended for registered attendees only, and is
subjected to the IEEE Copyright Policy. Any other use is strongly forbidden.
Papers from this author
Leveraging Synthetic Subject Invariant EEG Signals for Zero Calibration BCI
Nik Khadijah Nik Aznan, Amir Atapour-Abarghouei, Stephen Bonner, Jason Connolly, Toby Breckon
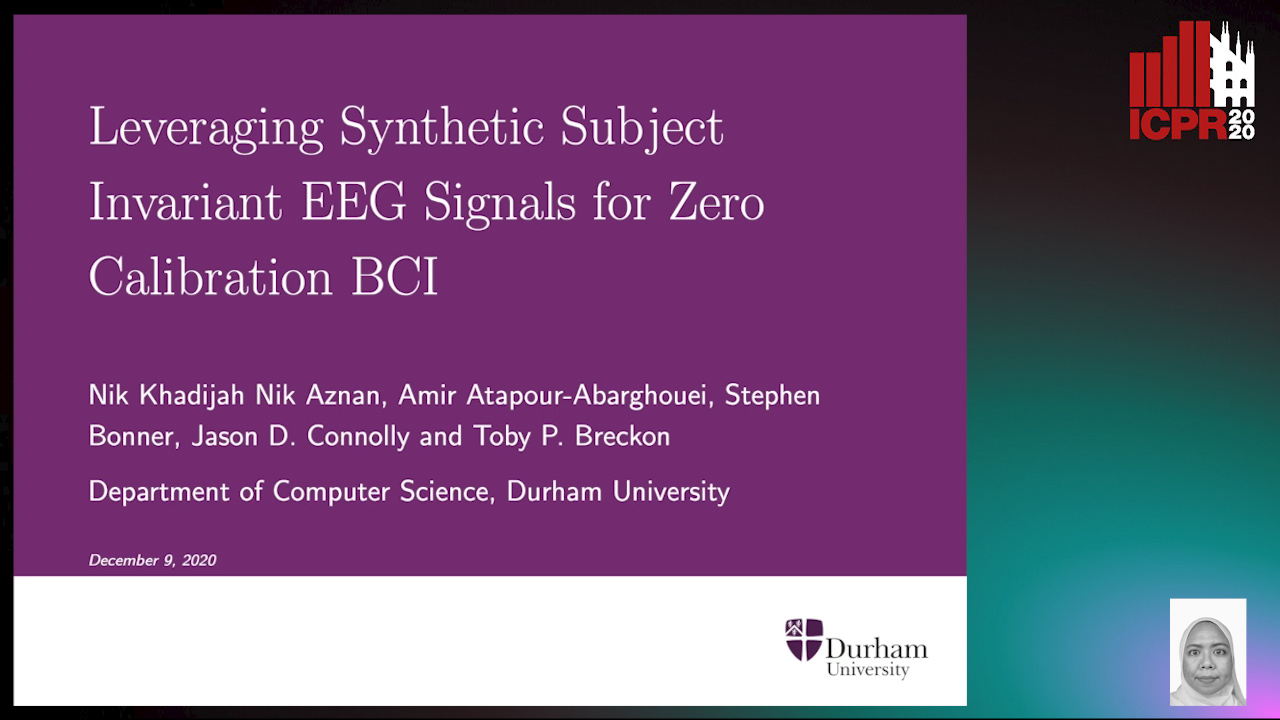
Auto-TLDR; SIS-GAN: Subject Invariant SSVEP Generative Adversarial Network for Brain-Computer Interface
Recently, substantial progress has been made in the area of Brain-Computer Interface (BCI) using modern machine learning techniques to decode and interpret brain signals. While Electroencephalography (EEG) has provided a non-invasive method of interfacing with a human brain, the acquired data is often heavily subject and session dependent. This makes seamless incorporation of such data into real-world applications intractable as the subject and session data variance can lead to long and tedious calibration requirements and cross-subject generalisation issues. Focusing on a Steady State Visual Evoked Potential (SSVEP) classification systems, we propose a novel means of generating highly-realistic synthetic EEG data invariant to any subject, session or other environmental conditions. Our approach, entitled the Subject Invariant SSVEP Generative Adversarial Network (SIS-GAN), produces synthetic EEG data from multiple SSVEP classes using a single network. Additionally, by taking advantage of a fixed-weight pre-trained subject classification network, we ensure that our generative model remains agnostic to subject-specific features and thus produces subject-invariant data that can be applied to new previously unseen subjects. Our extensive experimental evaluation demonstrates the efficacy of our synthetic data, leading to superior performance, with improvements of up to 16% in zero-calibration classification tasks when trained using our subject-invariant synthetic EEG signals.