Sriram Yenamandra
Paper download is intended for registered attendees only, and is
subjected to the IEEE Copyright Policy. Any other use is strongly forbidden.
Papers from this author
Learning Image Inpainting from Incomplete Images using Self-Supervision
Sriram Yenamandra, Rohit Kumar Jena, Ansh Khurana, Suyash Awate
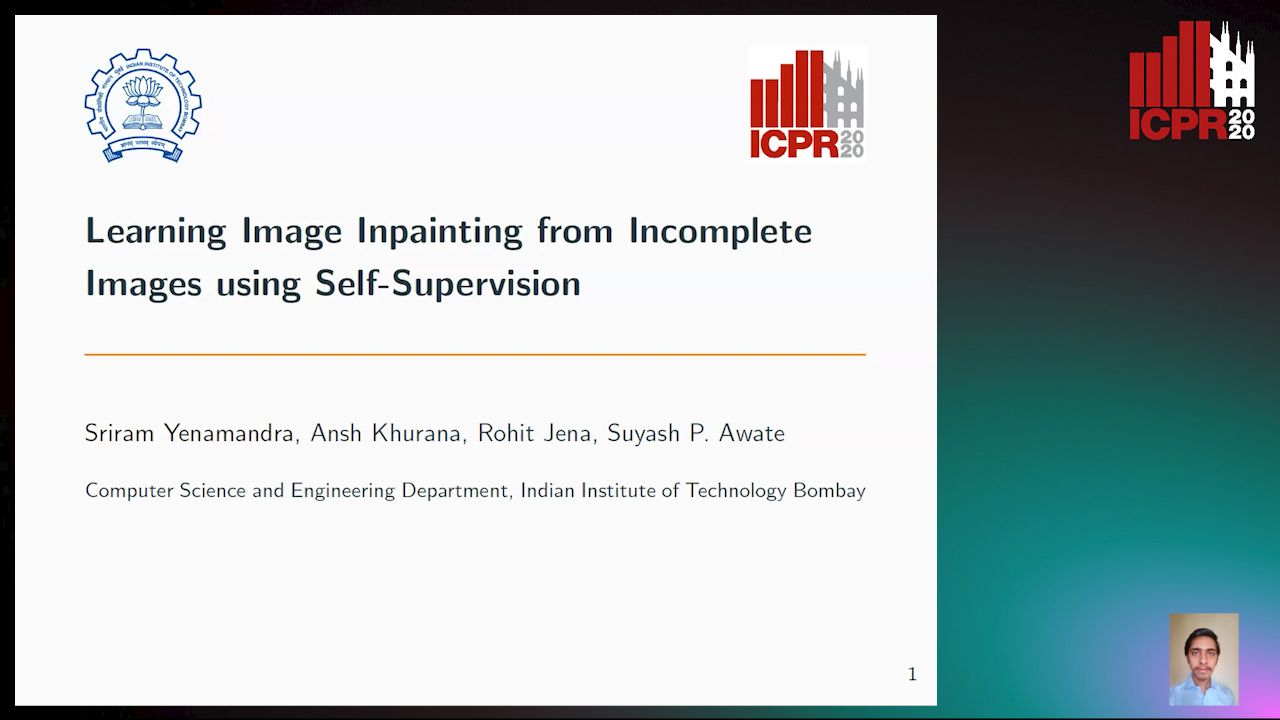
Auto-TLDR; Unsupervised Deep Neural Network for Semantic Image Inpainting
Abstract Slides Poster Similar
Current approaches for semantic image inpainting rely on deep neural networks (DNNs) that learn under full supervision, i.e., using a training set comprising pairs of (i)corrupted images with holes and (ii)corresponding uncorrupted images. However, for several real-world applications, obtaining large sets of uncorrupted images is challenging or infeasible. Current methods also rely on adversarial training involving min-max optimization that is prone to instability during learning. We propose a novel image-inpainting DNN framework that can learn in both completely unsupervised and semi-supervised modes. Moreover, our DNN learning formulation bypasses adversarial training and, thereby, lends itself to more stable training. Results on the publicly available CelebA dataset show that our method, even when learning unsupervisedly, outperforms the state of the art that learns with full supervision.