Huy Vo
Paper download is intended for registered attendees only, and is
subjected to the IEEE Copyright Policy. Any other use is strongly forbidden.
Papers from this author
Partial Monotone Dependence
Denis Khryashchev, Huy Vo, Robert Haralick
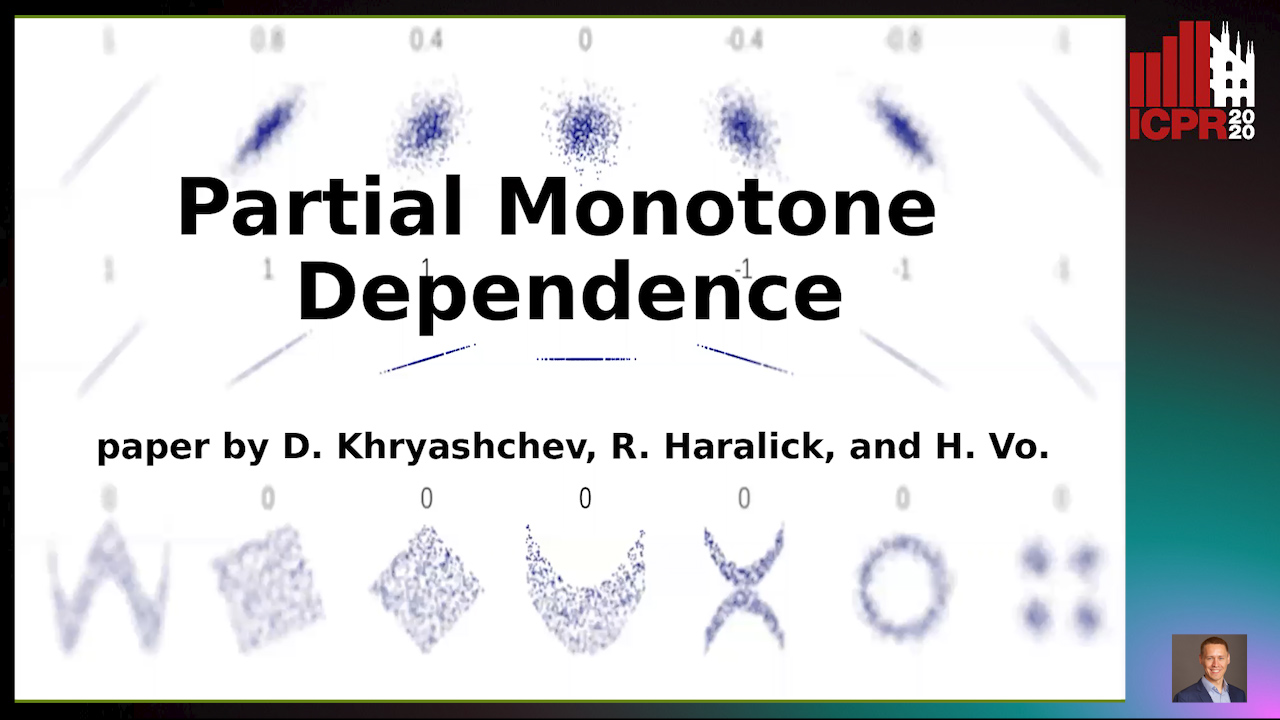
Auto-TLDR; Partially Monotone Autoregressive Correlation for Time Series Forecasting
We present a new measure of dependence suitable for time series forecasting: Partially Monotone Correlation (PMC) that generalizes Monotone Correlation. Unlike Monotone Correlation, the new measure of dependence uses piece-wise strictly monotone transformations that increase the value of the correlation coefficient. We explore its properties, its relationship with Monotone and Maximal Correlation, and present an algorithm that calculates it based on the Simultaneous Perturbation Stochastic Approximation method. We also demonstrate how to apply Partially Monotone Correlation for time series analysis and forecasting introducing Partially Monotone Autoregressive model (PMAR) of order 1. Its performance is then evaluated against the baseline of linear and non-linear autoregressive models (AR, LSTAR) on 150 time series produced from 3 datasets: Yellow Taxi pickups, Citi Bike pickups, and Cellular Network hits. Overall, PMAR model outperforms the baseline with the average sMAPE of about 1.7-4% lower.