David Peter
Paper download is intended for registered attendees only, and is
subjected to the IEEE Copyright Policy. Any other use is strongly forbidden.
Papers from this author
Resource-efficient DNNs for Keyword Spotting using Neural Architecture Search and Quantization
David Peter, Wolfgang Roth, Franz Pernkopf
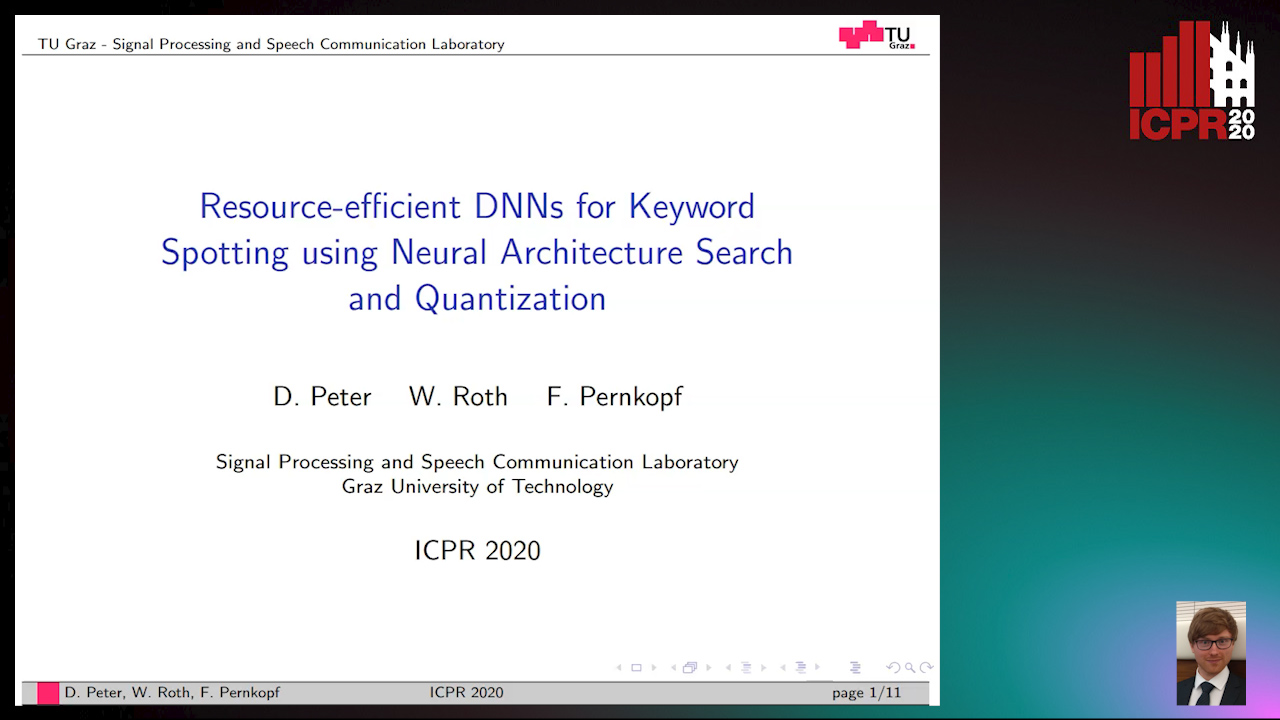
Auto-TLDR; Neural Architecture Search for Keyword Spotting in Limited Resource Environments
Abstract Slides Poster Similar
This paper introduces neural architecture search (NAS) for the automatic discovery of small models for keyword spotting (KWS) in limited resource environments. We employ a differentiable NAS approach to optimize the structure of convolutional neural networks (CNNs) to meet certain memory constraints for storing weights as well as constraints on the number of operations per inference. Using NAS only, we were able to obtain a highly efficient model with 95.6% accuracy on the Google speech commands dataset with 494.8 kB of memory usage and 19.6 million operations. Additionally, weight quantization is used to reduce the memory consumption even further. We show that weight quantization to low bit-widths (e.g. 1 bit) can be used without substantial loss in accuracy. By increasing the number of input features from 10 MFCC to 20 MFCC we were able to increase the accuracy to 96.6% at 340.1 kB of memory usage and 27.1 million operations.