Quan Nguyen
Paper download is intended for registered attendees only, and is
subjected to the IEEE Copyright Policy. Any other use is strongly forbidden.
Papers from this author
Improving Mix-And-Separate Training in Audio-Visual Sound Source Separation with an Object Prior
Quan Nguyen, Simone Frintrop, Timo Gerkmann, Mikko Lauri, Julius Richter
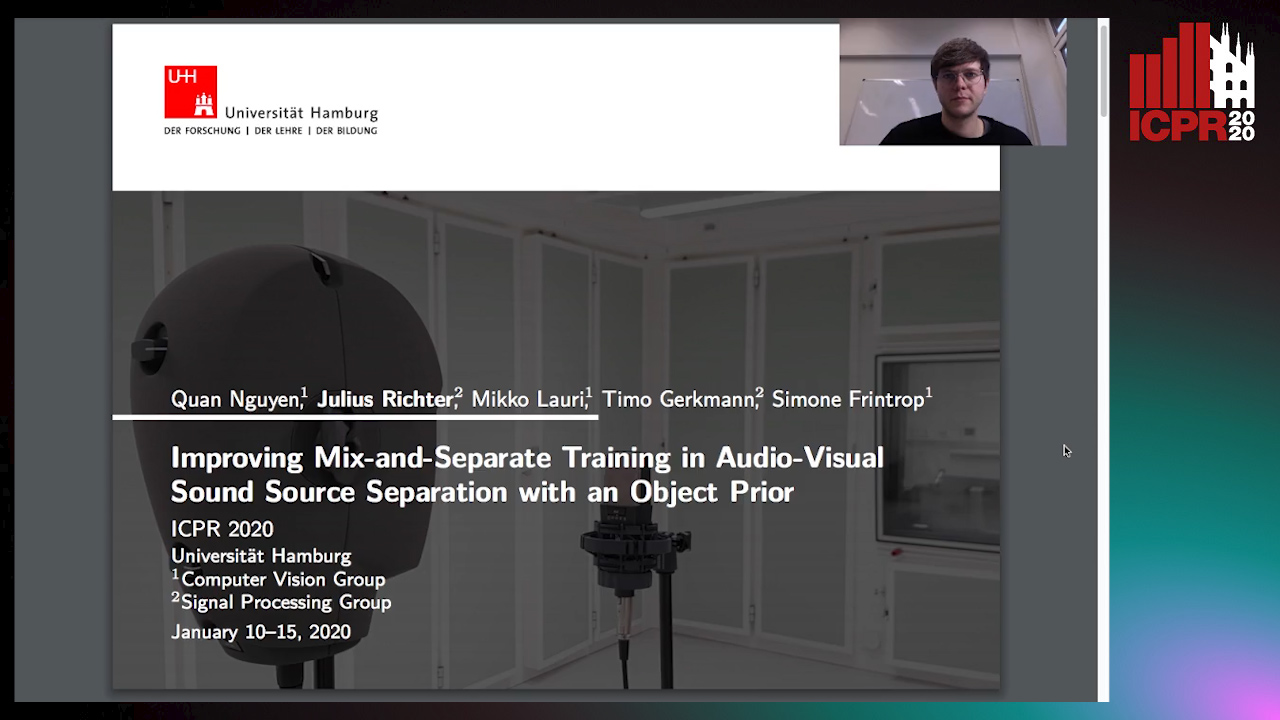
Auto-TLDR; Object-Prior: Learning the 1-to-1 correspondence between visual and audio signals by audio- visual sound source methods
The performance of an audio-visual sound source separation system is determined by its ability to separate audio sources given images of the sources and the audio mixture. The goal of this study is to investigate the ability to learn the mapping between the sounds and the images of instruments by audio- visual sound source separation methods based on the state-of-the- art PixelPlayer [1]. Theoretical and empirical analyses illustrate that the PixelPlayer is not properly trained to learn the 1-to- 1 correspondence between visual and audio signals during its mix-and-separate training process. Based on the insights from this analysis, a weakly-supervised method called Object-Prior is proposed and evaluated on two audio-visual datasets. The experimental results show that the proposed Object-Prior method outperforms the PixelPlayer and other baselines in the audio- visual sound source separation task. It is also more robust against asynchronized data, where the frame and the audio do not come from the same video, and recognizes musical instruments based on their sound with higher accuracy than the PixelPlayer. This indicates that learning the 1-to-1 correspondence between visual and audio features of an instrument improves the effectiveness of audio-visual sound source separation.