Mehmet Saygin Seyfioglu
Papers from this author
Leveraging Unlabeled Data for Glioma Molecular Subtype and Survival Prediction
Nicholas Nuechterlein, Beibin Li, Mehmet Saygin Seyfioglu, Sachin Mehta, Patrick Cimino, Linda Shapiro
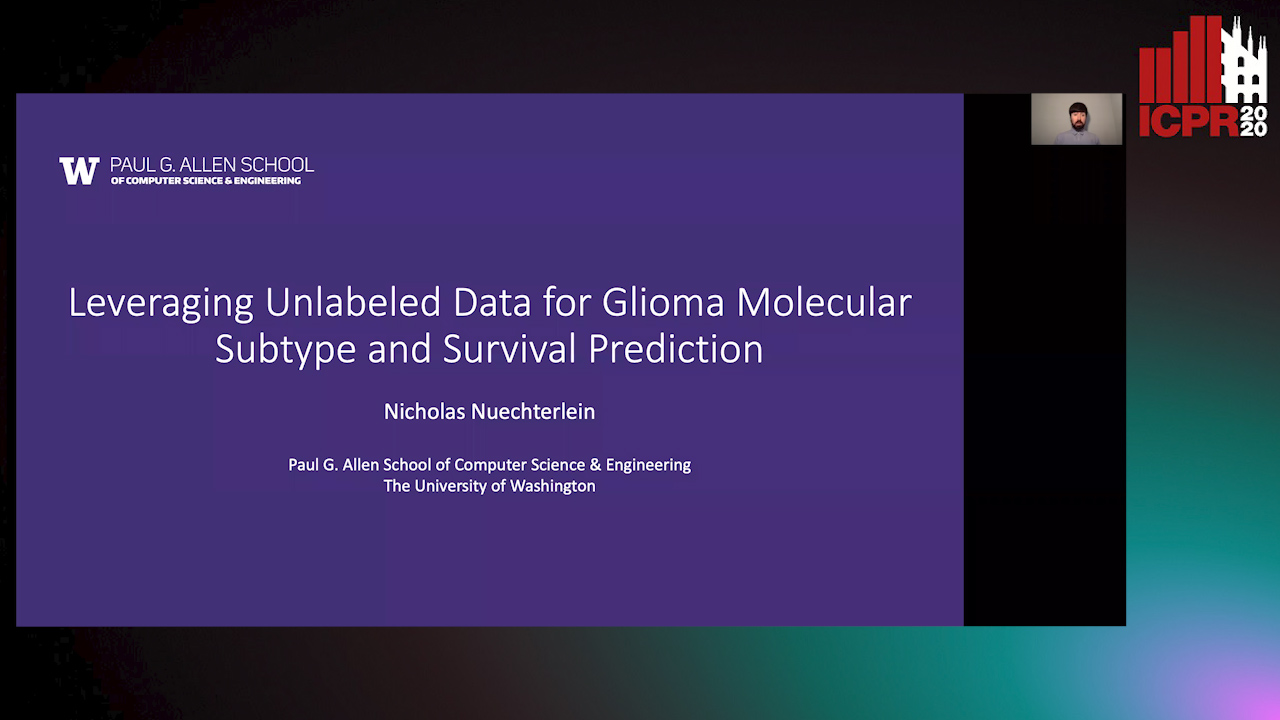
Auto-TLDR; Multimodal Brain Tumor Segmentation Using Unlabeled MR Data and Genomic Data for Cancer Prediction
Abstract Slides Poster Similar