Qingyuan Li
Paper download is intended for registered attendees only, and is
subjected to the IEEE Copyright Policy. Any other use is strongly forbidden.
Papers from this author
Fast, Accurate and Lightweight Super-Resolution with Neural Architecture Search
Chu Xiangxiang, Bo Zhang, Micheal Ma Hailong, Ruijun Xu, Jixiang Li, Qingyuan Li
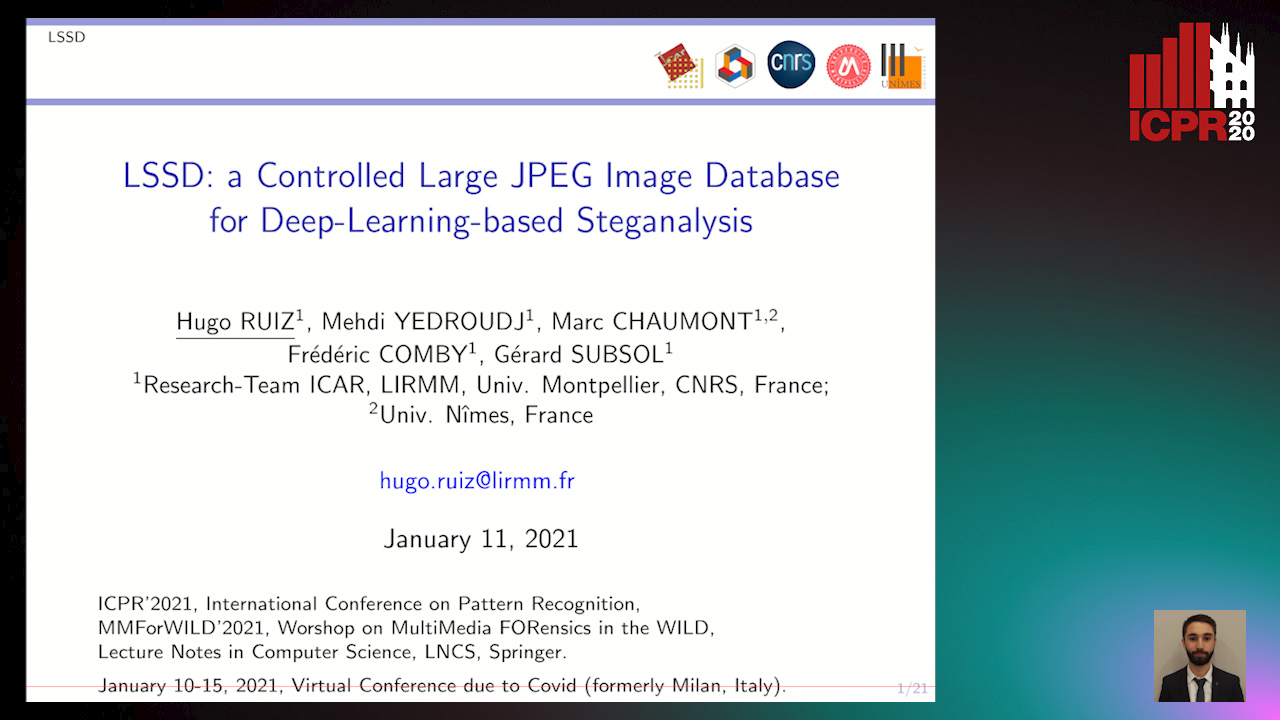
Auto-TLDR; Multi-Objective Neural Architecture Search for Super-Resolution
Abstract Slides Poster Similar
Deep convolutional neural networks demonstrate impressive results in the super-resolution domain. A series of studies concentrate on improving peak signal noise ratio (PSNR) by using much deeper layers, which are not friendly to constrained resources. Pursuing a trade-off between the restoration capacity and the simplicity of models is still non-trivial. Recent contributions are struggling to manually maximize this balance, while our work achieves the same goal automatically with neural architecture search. Specifically, we handle super-resolution with a multi-objective approach. We also propose an elastic search tactic at both micro and macro level, based on a hybrid controller that profits from evolutionary computation and reinforcement learning. Quantitative experiments help us to draw a conclusion that our generated models dominate most of the state-of-the-art methods with respect to the individual FLOPS.