Fatos Yarman Vural
Paper download is intended for registered attendees only, and is
subjected to the IEEE Copyright Policy. Any other use is strongly forbidden.
Papers from this author
Estimating Static and Dynamic Brain Networks by Kulback-Leibler Divergence from fMRI Data
Gonul Degirmendereli, Fatos Yarman Vural
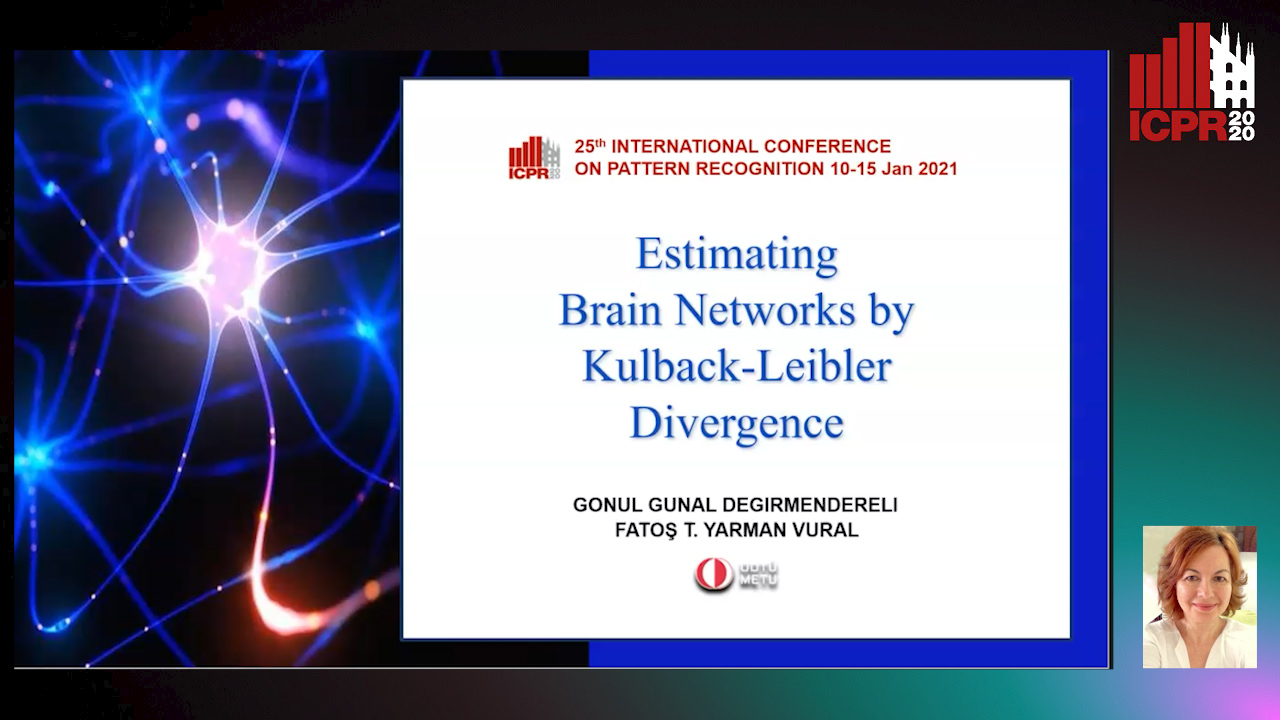
Auto-TLDR; A Novel method to estimate static and dynamic brain networks using Kulback- Leibler divergence using fMRI data
Representing the brain activities by networks is very crucial to understand the human brain and design brain-computer interfaces. This study proposes a novel method to estimate static and dynamic brain networks using Kulback- Leibler divergence. The suggested brain networks are based on the probability distributions of voxel intensity values measured by functional Magnetic Resonance Images (fMRI) recorded while the subjects perform a predefined cognitive task, namely complex problem-solving. We investigate the validity of the estimated brain networks by modeling and analyzing the different phases of the complex problem-solving processes of the human brain, namely planning and execution phases. The suggested computational network model is tested by Support Vector Machines. We observe that the network models can successfully discriminate the planning and execution phases of the complex problem-solving process with more than 90% accuracy, when the suggested dynamic networks, extracted from the fMRI data, are classified by Support Vector Machines.