Inti Zlobec
Paper download is intended for registered attendees only, and is
subjected to the IEEE Copyright Policy. Any other use is strongly forbidden.
Papers from this author
Classification of Intestinal Gland Cell-Graphs Using Graph Neural Networks
Linda Studer, Jannis Wallau, Heather Dawson, Inti Zlobec, Andreas Fischer
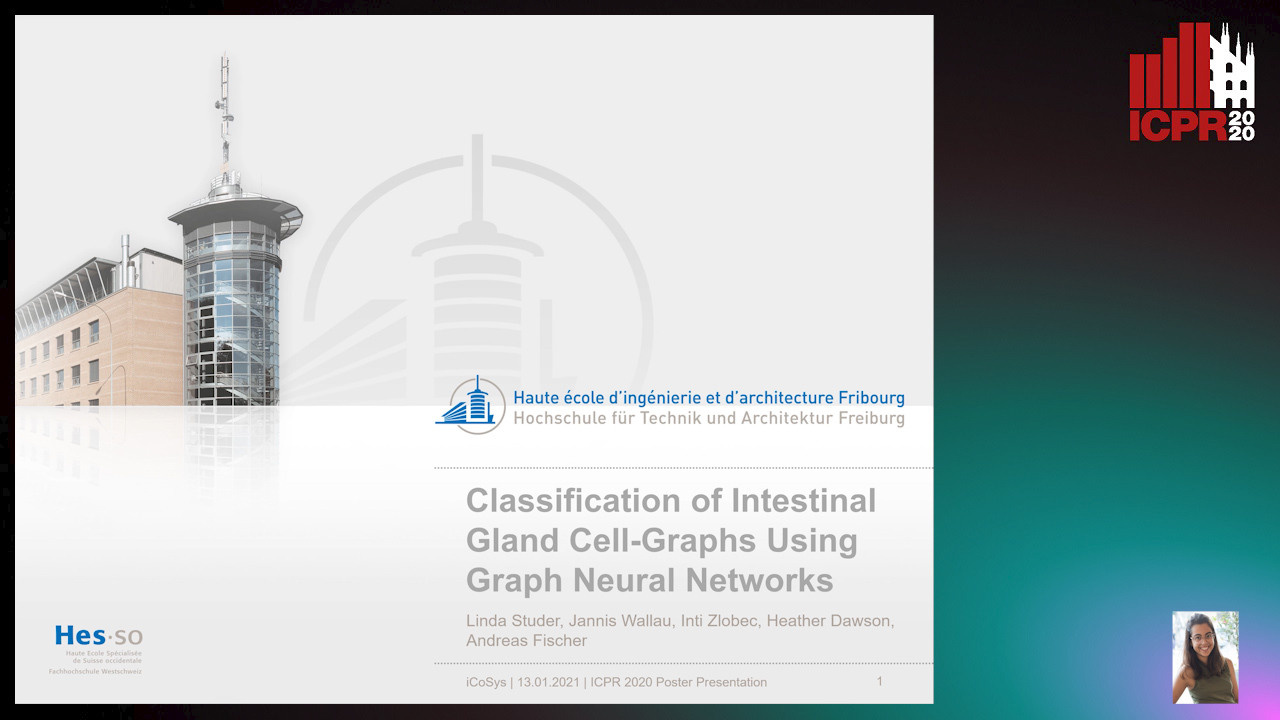
Auto-TLDR; Graph Neural Networks for Classification of Dysplastic Gland Glands using Graph Neural Networks
Abstract Slides Poster Similar
We propose to classify intestinal glands as normal or dysplastic using cell-graphs and graph-based deep learning methods. Dysplastic intestinal glands can lead to colorectal cancer, which is one of the three most common cancer types in the world. In order to assess the cancer stage and thus the treatment of a patient, pathologists analyse tissue samples of affected patients. Among other factors, they look at the changes in morphology of different tissues, such as the intestinal glands. Cell-graphs have a high representational power and can describe topological and geometrical properties of intestinal glands. However, classical graph-based methods have a high computational complexity and there is only a limited range of machine learning methods available. In this paper, we propose Graph Neural Networks (GNNs) as an efficient learning-based approach to classify cell-graphs. We investigate different variants of so-called Message Passing Neural Networks and compare them with a classical graph-based approach based on approximated Graph Edit Distance and k-nearest neighbours classifier. A promising classification accuracy of 94.1% is achieved by the proposed method on the pT1 Gland Graph dataset, which is an increase of 11.5% over the baseline result.