Kei Okada
Paper download is intended for registered attendees only, and is
subjected to the IEEE Copyright Policy. Any other use is strongly forbidden.
Papers from this author
Semi-Supervised Outdoor Image Generation Conditioned on Weather Signals
Sota Kawakami, Kei Okada, Naoko Nitta, Kazuaki Nakamura, Noboru Babaguchi
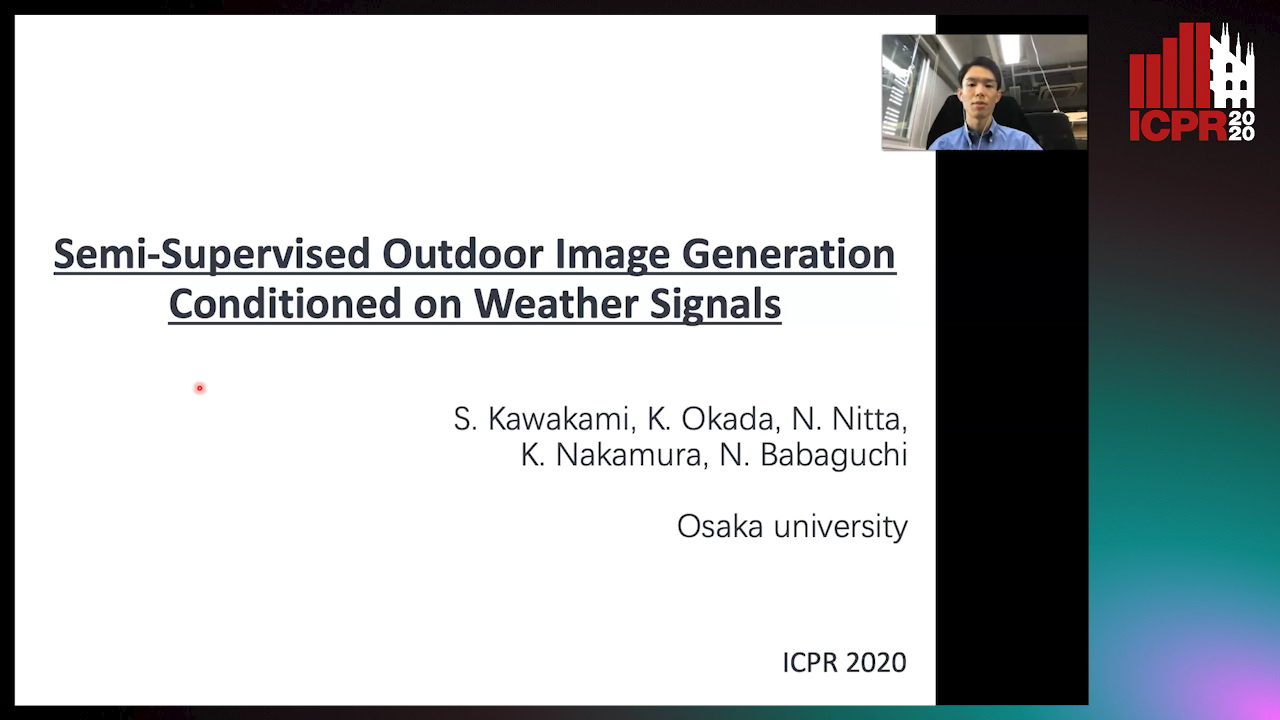
Auto-TLDR; Semi-supervised Generative Adversarial Network for Prediction of Weather Signals from Outdoor Images
Abstract Slides Poster Similar
In recent years, various types of sensors observe the real world. Especially, weather sensors are densely installed all over the world to observe current weather situations at various places. However, weather signals such as the temperature or humidity obtained by weather sensors are intuitively difficult for humans to understand. On the other hand, images captured by typical RGB cameras can tell weather situations at the captured places in a more comprehensible way for humans; however, cameras are only installed at limited places and are not necessarily open to public due to privacy issues. In order to solve this problem, the goal of our work is to generate images which can tell weather situations at arbitrary time and locations. This can be realized by using a conditional generative adversarial network architecture that takes an image and a condition to transform the image accordingly to the condition. Training such network requires a large number of image and condition pairs as the training data. Although weather signals can be easily collected from weather sensors, collecting their spatially and temporally synchronized outdoor images is not easy. Thus, we propose a semi-supervised method for training the image transformer. A relatively small number of pairs of an outdoor image and weather signals is collected, each from different web services, by considering their semantic consistency. The collected pairs are used to train a predictor for predicting weather signals from a given outdoor image. Then, the image transformer is trained by using a large number of pairs of an outdoor image and pseudo weather signals predicted by the predictor as the training data.