Sheng Liu
Paper download is intended for registered attendees only, and is
subjected to the IEEE Copyright Policy. Any other use is strongly forbidden.
Papers from this author
CT-UNet: An Improved Neural Network Based on U-Net for Building Segmentation in Remote Sensing Images
Huanran Ye, Sheng Liu, Kun Jin, Haohao Cheng
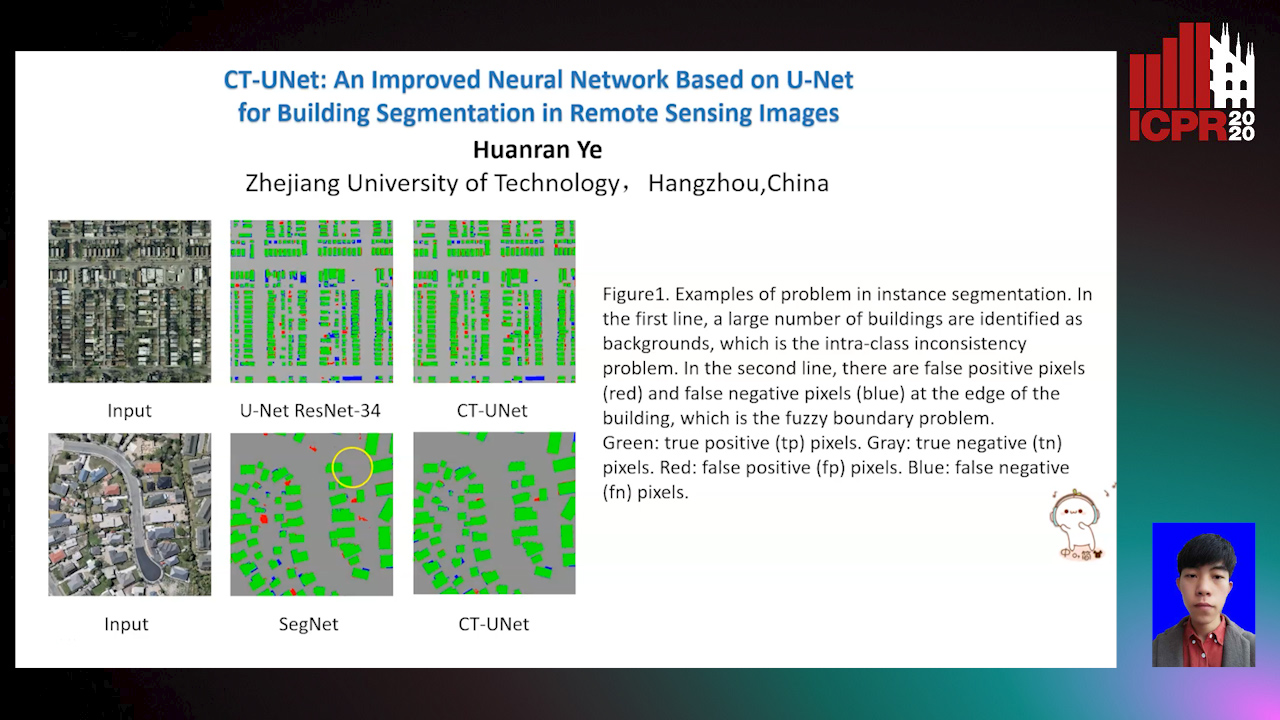
Auto-TLDR; Context-Transfer-UNet: A UNet-based Network for Building Segmentation in Remote Sensing Images
Abstract Slides Poster Similar
With the proliferation of remote sensing images, how to segment buildings more accurately in remote sensing images is a critical challenge. First, the high resolution leads to blurred boundaries in the extracted building maps. Second, the similarity between buildings and background results in intra-class inconsistency. To address these two problems, we propose an UNet-based network named Context-Transfer-UNet (CT-UNet). Specifically, we design Dense Boundary Block (DBB). Dense Block utilizes reuse mechanism to refine features and increase recognition capabilities. Boundary Block introduces the low-level spatial information to solve the fuzzy boundary problem. Then, to handle intra-class inconsistency, we construct Spatial Channel Attention Block (SCAB). It combines context space information and selects more distinguishable features from space and channel. Finally, we propose a novel loss function to enhance the purpose of loss by adding evaluation indicator. Based on our proposed CT-UNet, we achieve 85.33% mean IoU on the Inria dataset and 91.00% mean IoU on the WHU dataset, which outperforms our baseline (U-Net ResNet-34) by 3.76% and Web-Net by 2.24%.