Gözde Bozdağı Akar
Paper download is intended for registered attendees only, and is
subjected to the IEEE Copyright Policy. Any other use is strongly forbidden.
Papers from this author
Exploiting Local Indexing and Deep Feature Confidence Scores for Fast Image-To-Video Search
Savas Ozkan, Gözde Bozdağı Akar
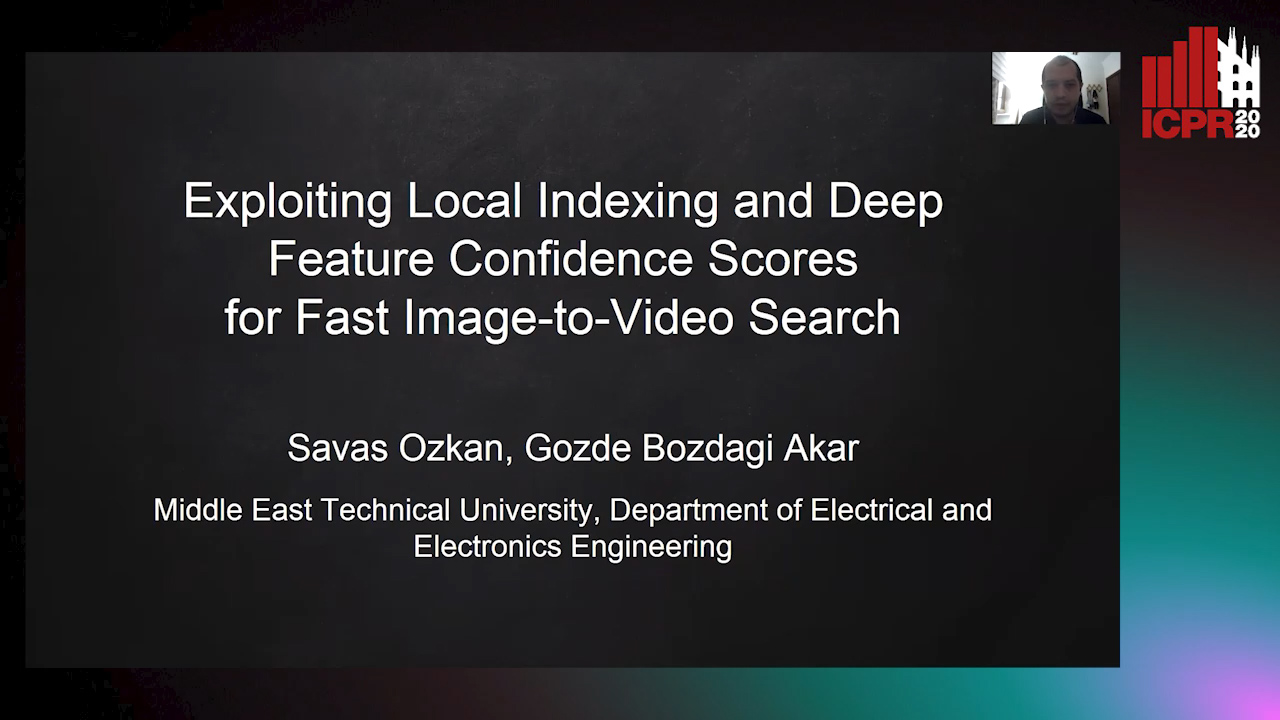
Auto-TLDR; Fast and Robust Image-to-Video Retrieval Using Local and Global Descriptors
Abstract Slides Poster Similar
Cost-effective visual representation and fast query-by-example search are two challenging goals hat should be provided for web-scale visual retrieval task on a moderate hardware. In this paper, we introduce a fast yet robust method that ensures both of these goals by obtaining the state-of-the-art results for an image-to-video search scenario. To this end, we present important enhancements to commonly used indexing and visual representation techniques by promoting faster, better and more moderate retrieval performance. We also boost the effectiveness of the method for visual distortion by exploiting the individual decision results of local and global descriptors in the query time. By this way, local content descriptors effectively represent copied / duplicated scenes with large geometric deformations, while global descriptors for near duplicate and semantic searches are more practical. Experiments are conducted on the large-scale Stanford I2V dataset. The experimental results show that the method is effective in terms of complexity and query processing time for large-scale visual retrieval scenarios, even if local and global representations are used together. In addition, the proposed method is fairly accurate and achieves state-of-the-art performance based on the mAP score of the dataset. Lastly, we report additional mAP scores after updating the ground annotations obtained by the retrieval results of the proposed method showing more clearly the actual performance.