Haoxue Wu
Paper download is intended for registered attendees only, and is
subjected to the IEEE Copyright Policy. Any other use is strongly forbidden.
Papers from this author
Exemplar Guided Cross-Spectral Face Hallucination Via Mutual Information Disentanglement
Haoxue Wu, Huaibo Huang, Aijing Yu, Jie Cao, Zhen Lei, Ran He
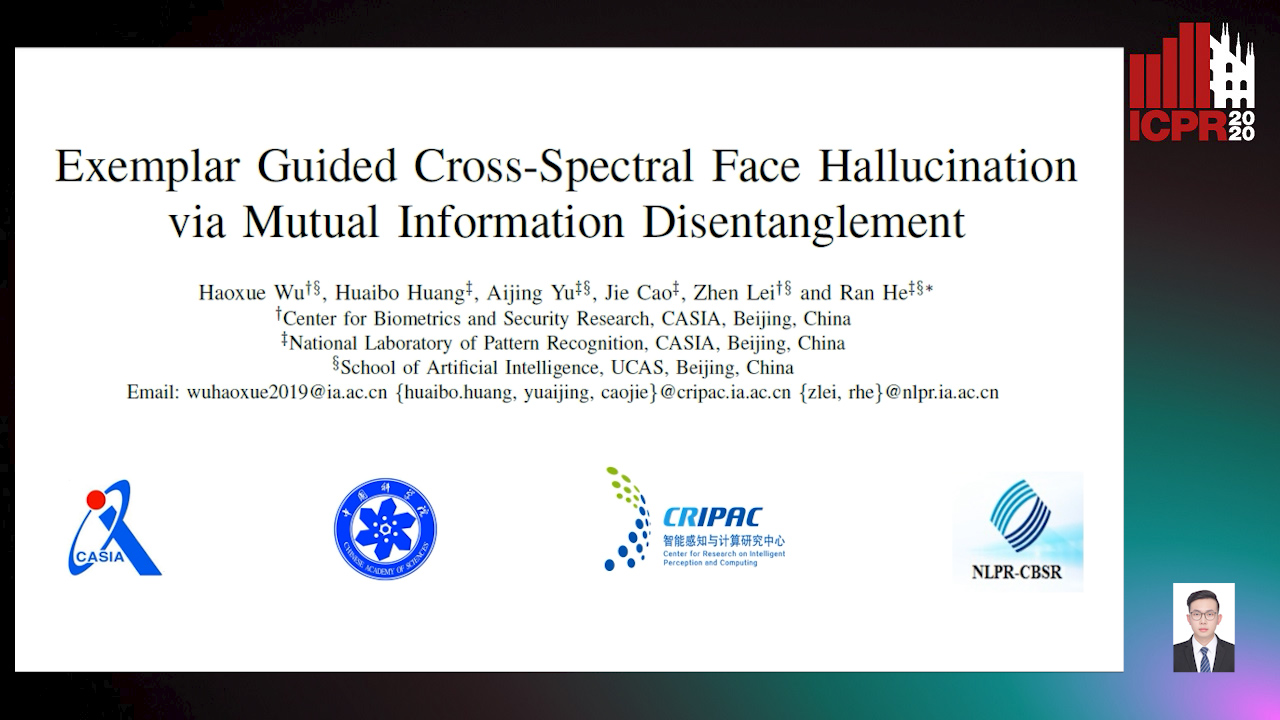
Auto-TLDR; Exemplar Guided Cross-Spectral Face Hallucination with Structural Representation Learning
Abstract Slides Poster Similar
Recently, many Near infrared-visible (NIR-VIS) heterogeneous face recognition (HFR) methods have been proposed in the community. But it remains a challenging problem because of the sensing gap along with large pose variations. In this paper, we propose an Exemplar Guided Cross-Spectral Face Hallucination (EGCH) to reduce the domain discrepancy through disentangled representation learning. For each modality, EGCH contains a spectral encoder as well as a structure encoder to disentangle spectral and structure representation, respectively. It also contains a traditional generator that reconstructs the input from the above two representations, and a structure generator that predicts the facial parsing map from the structure representation. Besides, mutual information minimization and maximization are conducted to boost disentanglement and make representations adequately expressed. Then the translation is built on structure representations between two modalities. Provided with the transformed NIR structure representation and original VIS spectral representation, EGCH is capable to produce high-fidelity VIS images that preserve the topology structure of the input NIR while transfer the spectral information of an arbitrary VIS exemplar. Extensive experiments demonstrate that the proposed method achieves more promising results both qualitatively and quantitatively than the state-of-the-art NIR-VIS methods.