Raoul Heese
Paper download is intended for registered attendees only, and is
subjected to the IEEE Copyright Policy. Any other use is strongly forbidden.
Papers from this author
Adaptive Sampling of Pareto Frontiers with Binary Constraints Using Regression and Classification
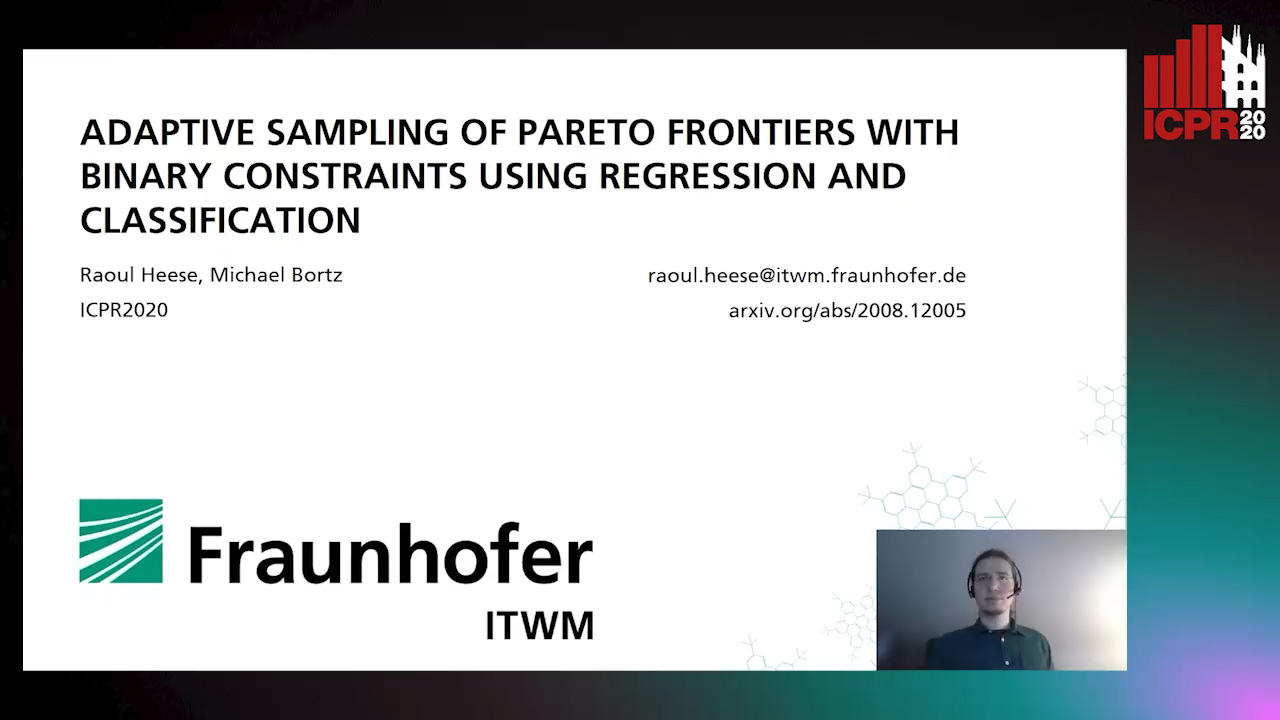
Auto-TLDR; Adaptive Optimization for Black-Box Multi-Objective Optimizing Problems with Binary Constraints
We present a novel adaptive optimization algorithm for black-box multi-objective optimization problems with binary constraints on the foundation of Bayes optimization. Our method is based on probabilistic regression and classification models, which act as a surrogate for the optimization goals and allow us to suggest multiple design points at once in each iteration. The proposed acquisition function is intuitively understandable and can be tuned to the demands of the problems at hand. We also present a novel ellipsoid truncation method to speed up the expected hypervolume calculation in a straightfoward way for regression models with a normal probability density. We benchmark our approach with an evolutionary algorithm on multiple test problems.