Vincent Parret
Paper download is intended for registered attendees only, and is
subjected to the IEEE Copyright Policy. Any other use is strongly forbidden.
Papers from this author
Semi-Supervised Deep Learning Techniques for Spectrum Reconstruction
Adriano Simonetto, Vincent Parret, Alexander Gatto, Piergiorgio Sartor, Pietro Zanuttigh
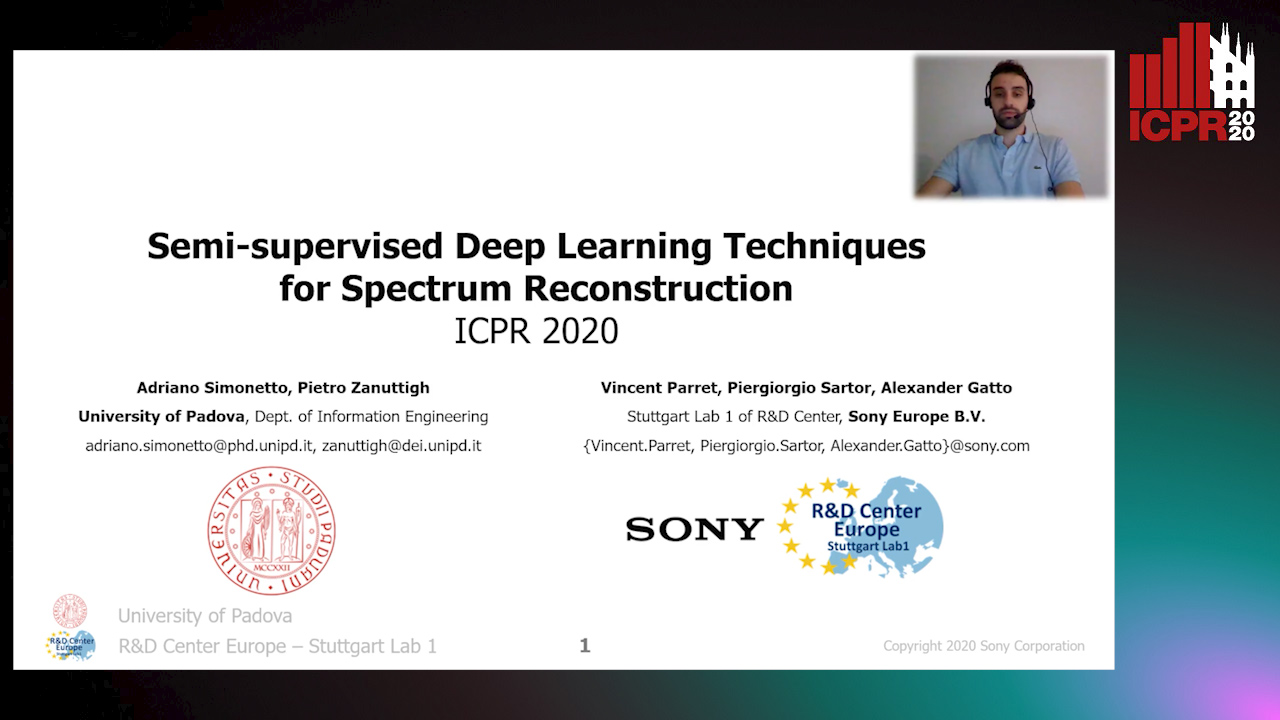
Auto-TLDR; hyperspectral data estimation from RGB data using semi-supervised learning
Abstract Slides Poster Similar
State-of-the-art approaches for the estimation of hyperspectral images (HSI) from RGB data are mostly based on deep learning techniques but due to the lack of training data their performances are limited to uncommon scenarios where a large hyperspectral database is available. In this work we present a family of novel deep learning schemes for hyperspectral data estimation able to work when the hyperspectral information at our disposal is limited. Firstly, we introduce a learning scheme exploiting a physical model based on the backward mapping to the RGB space and total variation regularization that can be trained with a limited amount of HSI images. Then, we propose a novel semi-supervised learning scheme able to work even with just a few pixels labeled with hyperspectral information. Finally, we show that the approach can be extended to a transfer learning scenario. The proposed techniques allow to reach impressive performances while requiring only some HSI images or just a few pixels for the training.