Cesare Alippi
Paper download is intended for registered attendees only, and is
subjected to the IEEE Copyright Policy. Any other use is strongly forbidden.
Papers from this author
PIF: Anomaly detection via preference embedding
Filippo Leveni, Luca Magri, Giacomo Boracchi, Cesare Alippi
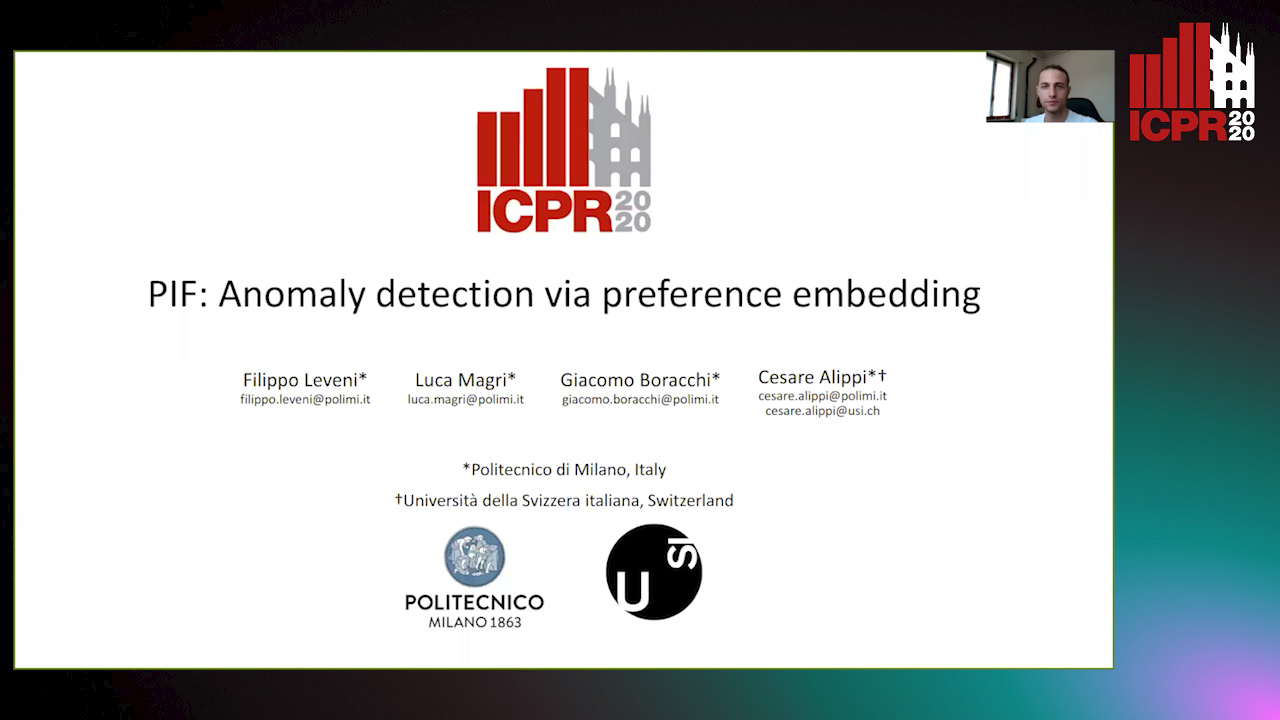
Auto-TLDR; PIF: Anomaly Detection with Preference Embedding for Structured Patterns
Abstract Slides Poster Similar
We address the problem of detecting anomalies with respect to structured patterns. To this end, we conceive a novel anomaly detection method called PIF, that combines the advantages of adaptive isolation methods with the flexibility of preference embedding. Specifically, we propose to embed the data in a high dimensional space where an efficient tree-based method, PI-FOREST, is employed to compute an anomaly score. Experiments on synthetic and real datasets demonstrate that PIF favorably compares with state-of-the-art anomaly detection techniques, and confirm that PI-FOREST is better at measuring arbitrary distances and isolate points in the preference space.