Shabnam Sadegharmaki
Paper download is intended for registered attendees only, and is
subjected to the IEEE Copyright Policy. Any other use is strongly forbidden.
Papers from this author
FashionGraph: Understanding Fashion Data Using Scene Graph Generation
Shabnam Sadegharmaki, Marc A. Kastner, Shin'Ichi Satoh
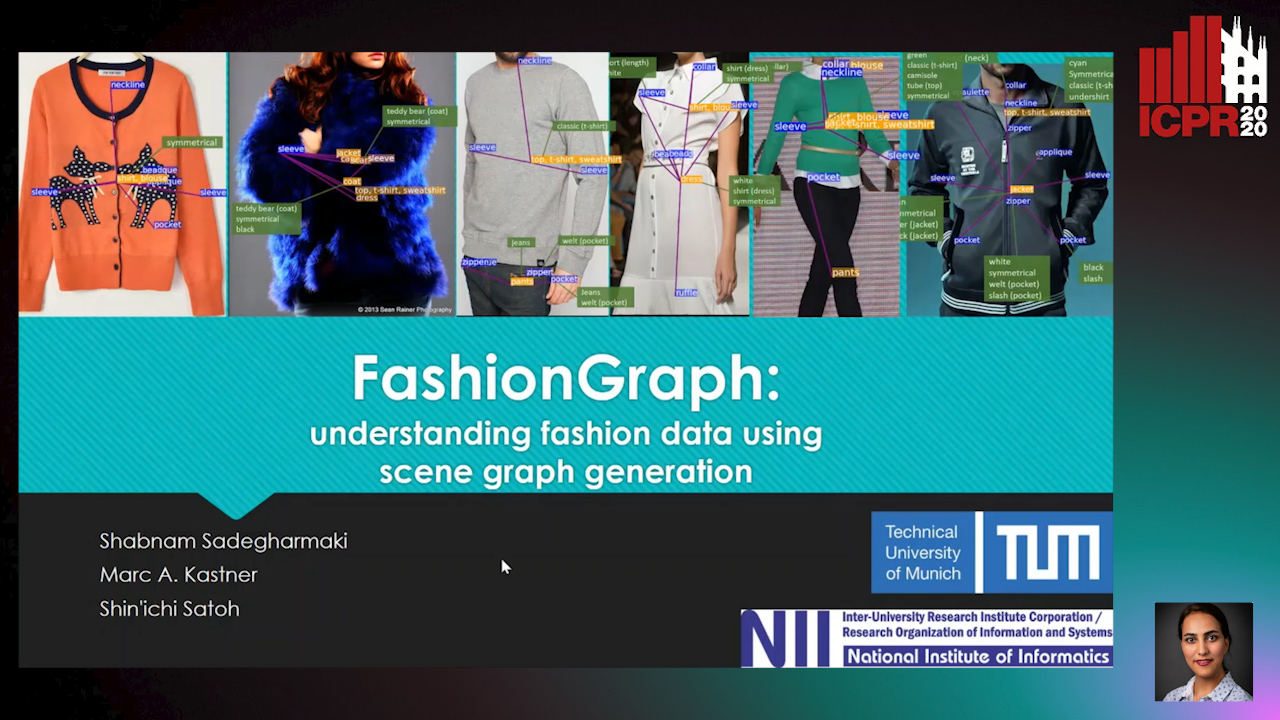
Auto-TLDR; Exploiting Scene Graph Knowledge for Fashion Applications
Fashion analysis is an attractive domain for vision research due to its direct applications in e-commerce contexts. However, fashion datasets are commonly rather demanding, as both objects and attributes tend to be fine-grained and thus result in very long-tailed datasets. Furthermore, relationships between objects and attributes are often dense, but are crucial for the performance of fashion applications. In this paper, we propose to generate scene graphs for existing fashion datasets. By detecting relationships between fashion objects, their parts, and their attributes we gain a better understanding of the scenes. As no current fashion dataset provides scene graphs, we generate relationships between fashion objects from existing annotations. The output is post-processed and filtered to generate a meaningful scene graph for each image. In the experiments we can show existing applications like image retrieval benefiting from the scene graph understanding. We first evaluate the accuracy of the generated scene graphs. Then, we employ scene graphs to fashion image retrieval in order to showcase their performance in real applications. The results show various benefits for fashion applications by exploiting scene graph knowledge. The sources and model for the proposed method will be made available after publication.