Susumu Kubota
Paper download is intended for registered attendees only, and is
subjected to the IEEE Copyright Policy. Any other use is strongly forbidden.
Papers from this author
Sample-Dependent Distance for 1 : N Identification Via Discriminative Feature Selection
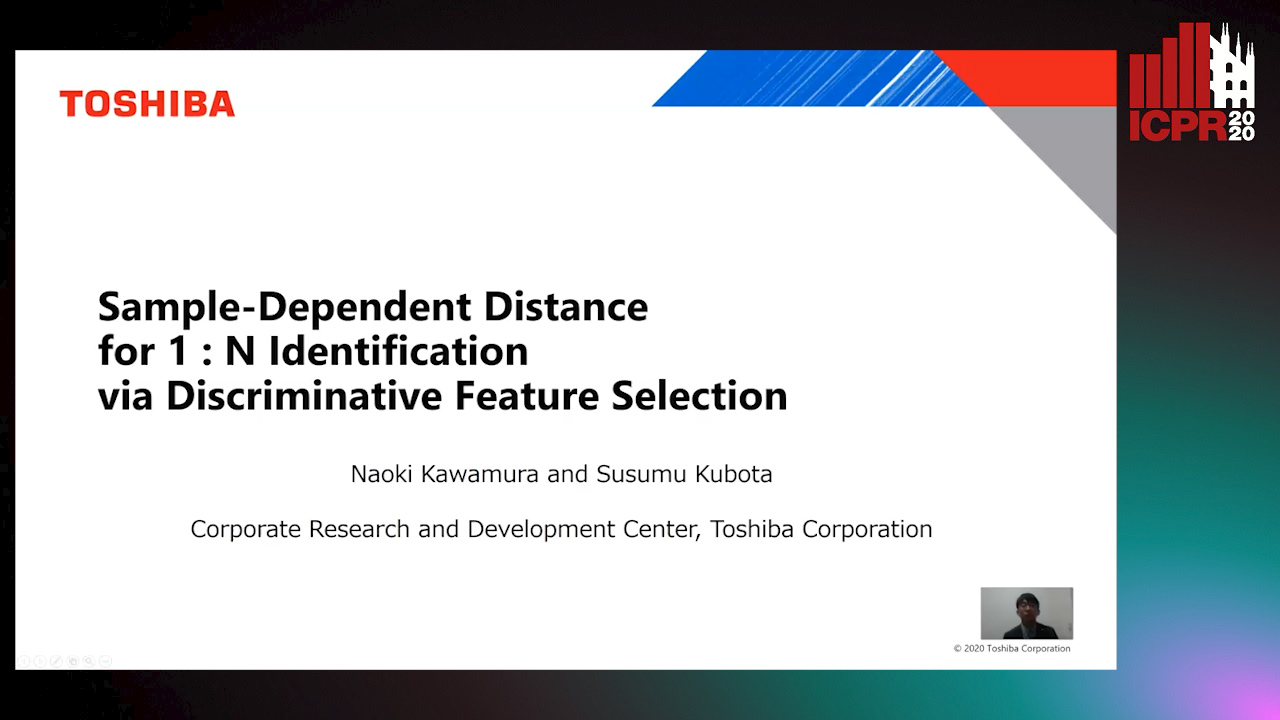
Auto-TLDR; Feature Selection Mask for 1:N Identification Problems with Binary Features
Abstract Slides Poster Similar
We focus on 1:N identification problems with binary features. Most multiclass classification methods, including identification and verification methods, use a shared metric space in which distances between samples are measured regardless of their identities. This is because dedicated metric spaces learned for each identity in the training set are of little use for the test set. In 1:N identification problems, however, gallery samples contain rich information about the test domain. Given a sample and its neighbors in the gallery set, we propose a method for calculating a discriminative feature selection mask that is used as a sample-dependent distance metric. Experiments on several re-identification datasets show that the proposed method enhances the performance of state-of-the-art feature extractors.