Nilay Pande
Paper download is intended for registered attendees only, and is
subjected to the IEEE Copyright Policy. Any other use is strongly forbidden.
Papers from this author
Generative Deep-Neural-Network Mixture Modeling with Semi-Supervised MinMax+EM Learning
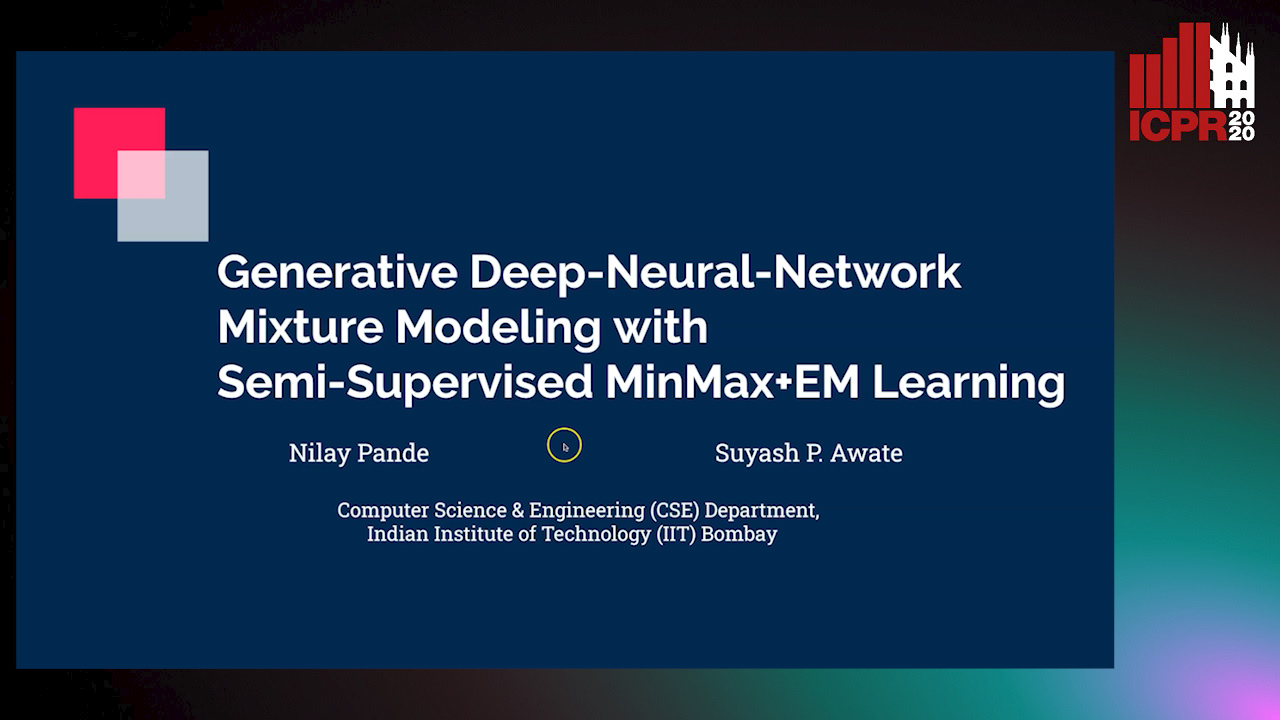
Auto-TLDR; Semi-supervised Deep Neural Networks for Generative Mixture Modeling and Clustering
Abstract Slides Poster Similar
Deep neural networks (DNNs) for generative mixture modeling typically rely on unsupervised learning that employs hard clustering schemes, or variational learning with loose / approximate bounds, or under-regularized modeling. We propose a novel statistical framework for a DNN mixture model using a single generative adversarial network. Our learning formulation proposes a novel data-likelihood term relying on a well-regularized / constrained Gaussian mixture model in the latent space along with a prior term on the DNN weights. Our min-max learning increases the data likelihood using a tight variational lower bound using expectation maximization (EM). We leverage our min-max EM learning scheme for semi-supervised learning. Results on three real-world datasets demonstrate the benefits of our compact modeling and learning formulation over the state of the art for mixture modeling and clustering.