Xin Yang
Paper download is intended for registered attendees only, and is
subjected to the IEEE Copyright Policy. Any other use is strongly forbidden.
Papers from this author
A Spectral Clustering on Grassmann Manifold Via Double Low Rank Constraint
Xinglin Piao, Yongli Hu, Junbin Gao, Yanfeng Sun, Xin Yang, Baocai Yin
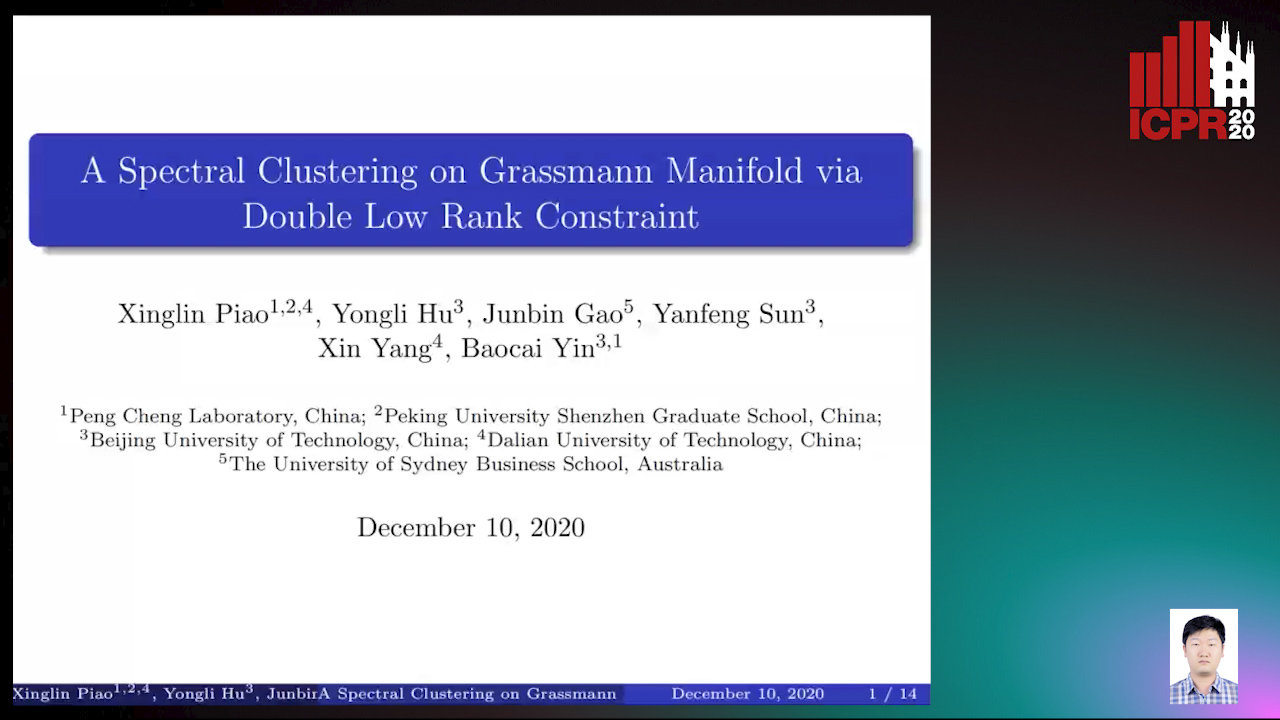
Auto-TLDR; Double Low Rank Representation for High-Dimensional Data Clustering on Grassmann Manifold
High-dimension data clustering is a fundamental topic in machine learning and data mining areas. In recent year, researchers have proposed a series of effective methods based on Low Rank Representation (LRR) which could explore low-dimension subspace structure embedded in original data effectively. The traditional LRR methods usually treat original data as samples in Euclidean space. They generally adopt linear metric to measure the distance between two data. However, high-dimension data (such as video clip or imageset) are always considered as non-linear manifold data such as Grassmann manifold. Therefore, the traditional linear Euclidean metric would be no longer suitable for these special data. In addition, traditional LRR clustering method always adopt nuclear norm as low rank constraint which would lead to suboptimal solution and decrease the clustering accuracy. In this paper, we proposed a new low rank method on Grassmann manifold for high-dimension data clustering task. In the proposed method, a double low rank representation approach is proposed by combining the nuclear norm and bilinear representation for better construct the representation matrix. The experimental results on several public datasets show that the proposed method outperforms the state-of-the-art clustering methods.