Naoto Inoue
Paper download is intended for registered attendees only, and is
subjected to the IEEE Copyright Policy. Any other use is strongly forbidden.
Papers from this author
Augmented Cyclic Consistency Regularization for Unpaired Image-To-Image Translation
Takehiko Ohkawa, Naoto Inoue, Hirokatsu Kataoka, Nakamasa Inoue
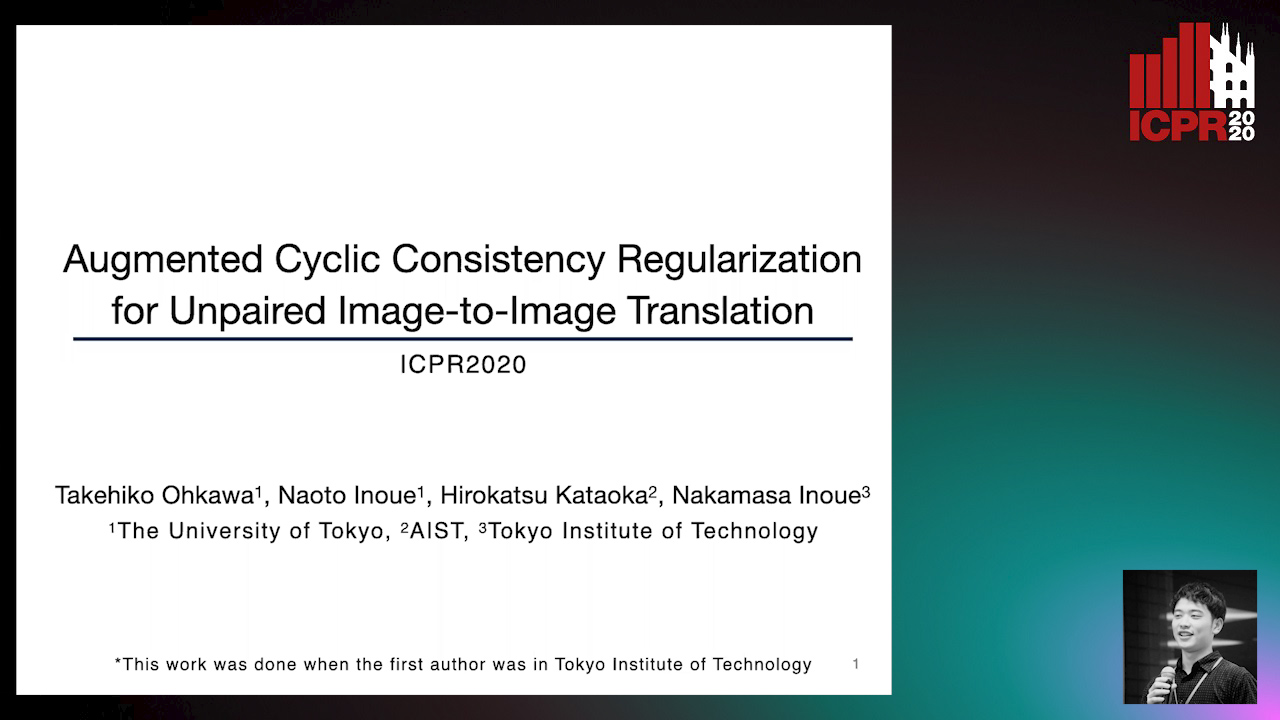
Auto-TLDR; Augmented Cyclic Consistency Regularization for Unpaired Image-to-Image Translation
Abstract Slides Poster Similar
Unpaired image-to-image (I2I) translation has received considerable attention in pattern recognition and computer vision because of recent advancements in generative adversarial networks (GANs). However, due to the lack of explicit supervision, unpaired I2I models often fail to generate realistic images, especially in challenging datasets with different backgrounds and poses. Hence, stabilization is indispensable for real-world applications and GANs. Herein, we propose Augmented Cyclic Consistency Regularization (ACCR), a novel regularization method for unpaired I2I translation. Our main idea is to enforce consistency regularization originating from semi-supervised learning on the discriminators leveraging real, fake, reconstructed, and augmented samples. We regularize the discriminators to output similar predictions when fed pairs of original and perturbed images. We qualitatively clarify the generation property between unpaired I2I models and standard GANs, and explain why consistency regularization on fake and reconstructed samples works well. Quantitatively, our method outperforms the consistency regularized GAN (CR-GAN) in real-world translations and demonstrates efficacy against several data augmentation variants and cycle-consistent constraints.