Hongjing Niu
Paper download is intended for registered attendees only, and is
subjected to the IEEE Copyright Policy. Any other use is strongly forbidden.
Papers from this author
Interpreting the Latent Space of GANs Via Correlation Analysis for Controllable Concept Manipulation
Ziqiang Li, Rentuo Tao, Hongjing Niu, Bin Li
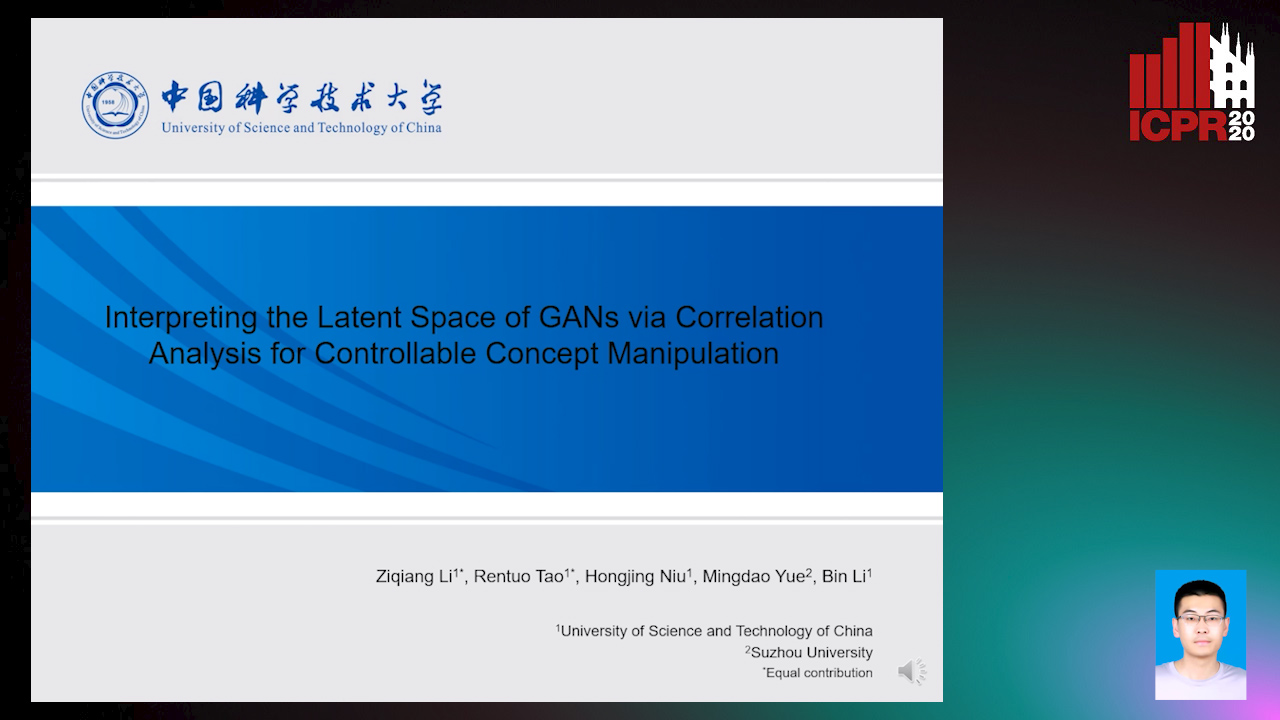
Auto-TLDR; Exploring latent space of GANs by analyzing correlation between latent variables and semantic contents in generated images
Abstract Slides Poster Similar
Generative adversarial nets (GANs) have been successfully applied in many fields like image generation, inpainting, super-resolution and drug discovery, etc., by now, the inner process of GANs is far from been understood. To get deeper insight of the intrinsic mechanism of GANs, in this paper, a method for interpreting the latent space of GANs by analyzing the correlation between latent variables and the corresponding semantic contents in generated images is proposed. Unlike previous methods that focus on dissecting models via feature visualization, the emphasis of this work is put on the variables in latent space, i.e. how the latent variables affect the quantitative analysis of generated results. Given a pretrained GAN model with weights fixed, the latent variables are intervened to analyze their effect on the semantic content in generated images. A set of controlling latent variables can be derived for specific content generation, and the controllable semantic content manipulation be achieved. The proposed method is testified on the datasets Fashion-MNIST and UT Zappos50K, experiment results show its effectiveness