Xinge You
Paper download is intended for registered attendees only, and is
subjected to the IEEE Copyright Policy. Any other use is strongly forbidden.
Papers from this author
Adaptive Matching of Kernel Means
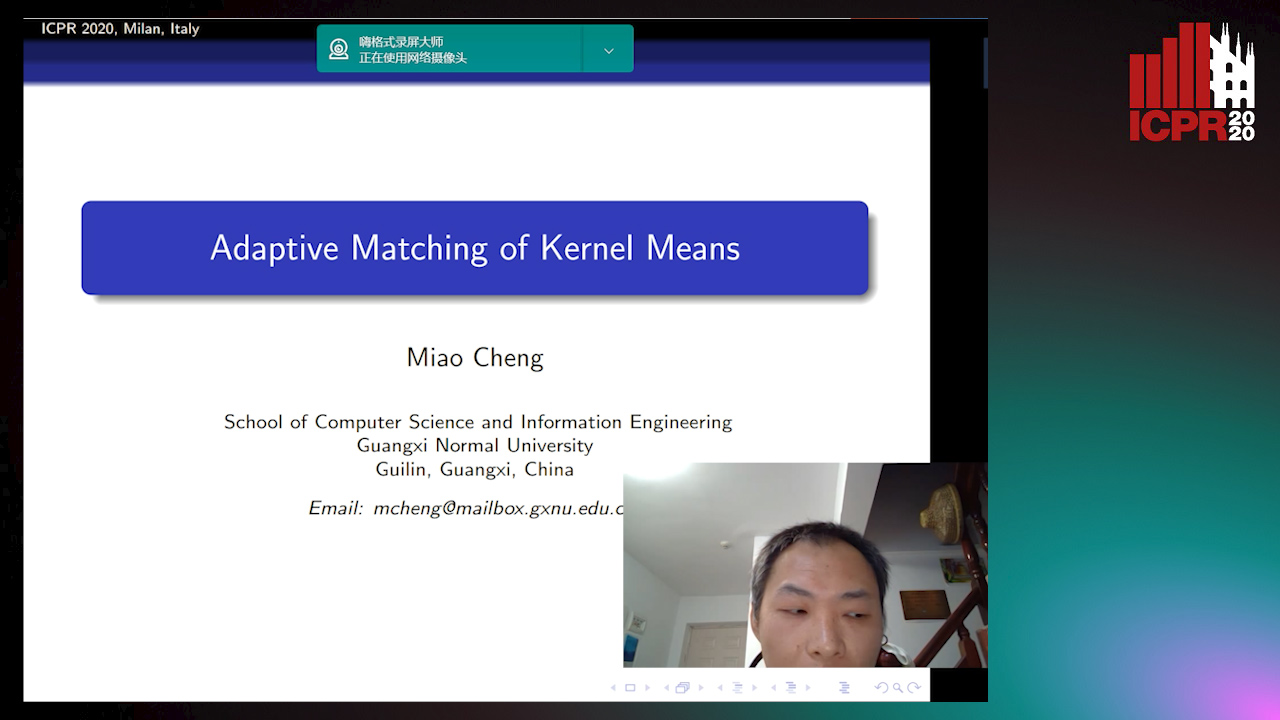
Auto-TLDR; Adaptive Matching of Kernel Means for Knowledge Discovery and Feature Learning
Abstract Slides Poster Similar
As a promising step, the performance of data analysis and feature learning are able to be improved if certain pattern matching mechanism is available. One of the feasible solutions can refer to the importance estimation of instances, and consequently, kernel mean matching (KMM) has become an important method for knowledge discovery and novelty detection in general. Furthermore, the existing KMM methods have focused on concrete learning frameworks. In this work, a novel approach to adaptive matching of kernel means is proposed, and selected data with high importance are adopted to achieve calculation efficiency with optimization. In addition, scalable learning can be conducted in proposed method as a generalized solution with appended data. The experimental results on a wide variety of real-world data sets demonstrate the proposed method is able to give outstanding performance compared with several state-of-the-art methods, while calculation efficiency can be preserved.