Tao Li
Paper download is intended for registered attendees only, and is
subjected to the IEEE Copyright Policy. Any other use is strongly forbidden.
Papers from this author
T-SVD Based Non-Convex Tensor Completion and Robust Principal Component Analysis
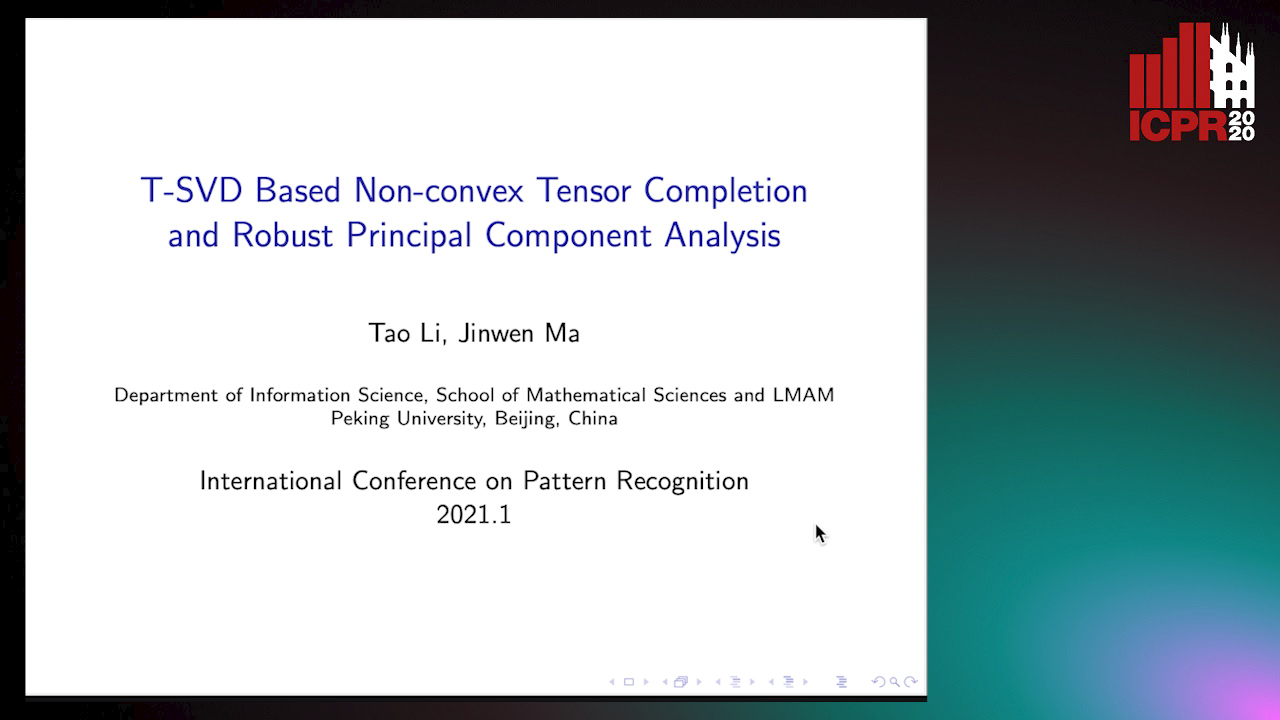
Auto-TLDR; Non-Convex tensor rank surrogate function and non-convex sparsity measure for tensor recovery
Abstract Slides Poster Similar
In this paper, we propose a novel non-convex tensor rank surrogate function and a novel non-convex sparsity measure. The basic idea is to sidestep the bias of $\ell_1-$norm by introducing the concavity. Furthermore, we employ this non-convex penalty in tensor recovery problems such as tensor completion and tensor robust principal component analysis. Due to the concavity, the parameters of these models are difficult to solve. To tackle this problem, we devise a majorization minimization algorithm that can optimize the upper bound of the original function in each iteration, and every sub-problem is solved by the alternating direction multiplier method. We also analyze the theoretical properties of the proposed algorithm. Finally, the experimental results on natural and hyperspectral images demonstrate the efficacy and efficiency of the proposed method.