Qiang Ji
Paper download is intended for registered attendees only, and is
subjected to the IEEE Copyright Policy. Any other use is strongly forbidden.
Papers from this author
Variational Deep Embedding Clustering by Augmented Mutual Information Maximization
Qiang Ji, Yanfeng Sun, Yongli Hu, Baocai Yin
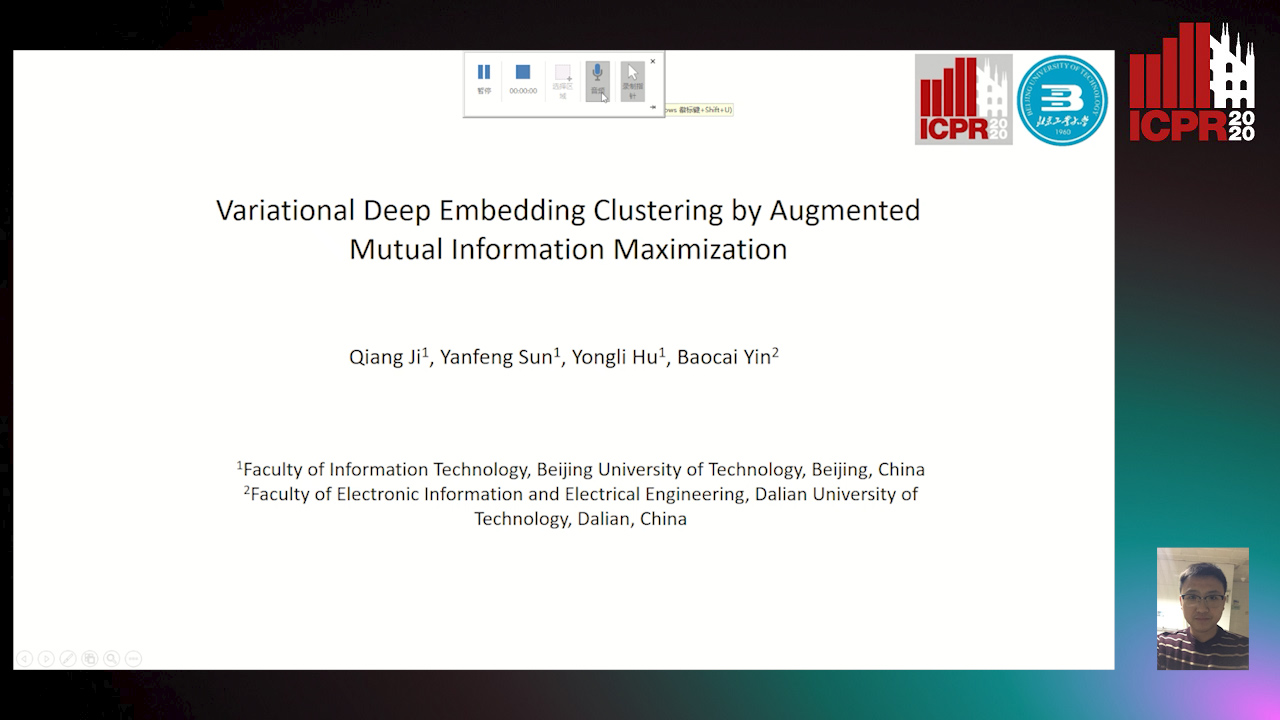
Auto-TLDR; Clustering by Augmented Mutual Information maximization for Deep Embedding
Abstract Slides Poster Similar
Clustering is a crucial but challenging task in pattern analysis and machine learning. Recent many deep clustering methods combining representation learning with cluster techniques emerged. These deep clustering methods mainly focus on the correlation among samples and ignore the relationship between samples and their representations. In this paper, we propose a novel end-to-end clustering framework, namely variational deep embedding clustering by augmented mutual information maximization (VCAMI). From the perspective of VAE, we prove that minimizing reconstruction loss is equivalent to maximizing the mutual information of the input and its latent representation. This provides a theoretical guarantee for us to directly maximize the mutual information instead of minimizing reconstruction loss. Therefore we proposed the augmented mutual information which highlights the uniqueness of the representations while discovering invariant information among similar samples. Extensive experiments on several challenging image datasets show that the VCAMI achieves good performance. we achieve state-of-the-art results for clustering on MNIST (99.5%) and CIFAR-10 (65.4%) to the best of our knowledge.