Roberto Roberto Ardon
Paper download is intended for registered attendees only, and is
subjected to the IEEE Copyright Policy. Any other use is strongly forbidden.
Papers from this author
Combining Similarity and Adversarial Learning to Generate Visual Explanation: Application to Medical Image Classification
Martin Charachon, Roberto Roberto Ardon, Celine Hudelot, Paul-Henry Cournède, Camille Ruppli
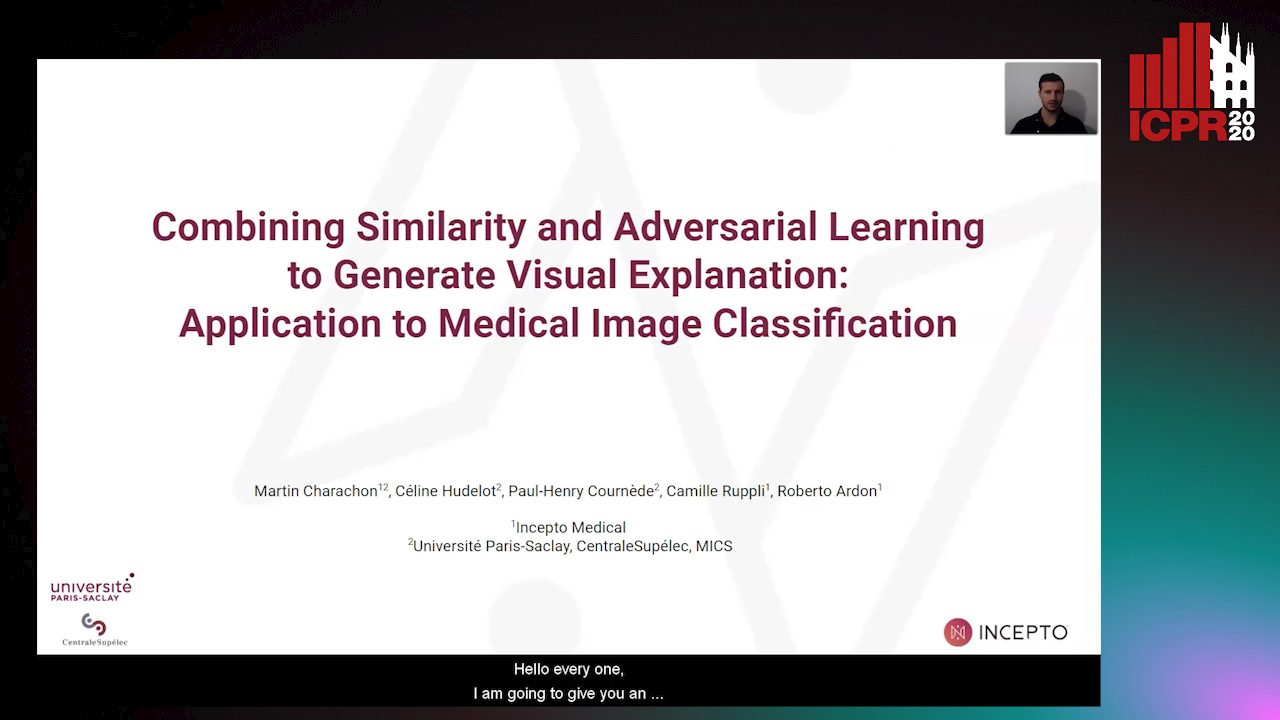
Auto-TLDR; Explaining Black-Box Machine Learning Models with Visual Explanation
Abstract Slides Poster Similar
Recently, due to their success and increasing applications, explaining the decision of black-box machine learning models has become a critical task. It is particularly the case in sensitive domains such as medical image interpretation. Various explanation approaches have been proposed in the literature, among which perturbation based approaches are very promising. Within this class of methods, we leverage a learning framework to produce our visual explanations method. From a given classifier, we train two generators to produce from an input image the so called similar and adversarial images. The similar (resp. adversarial) image shall be classified as (resp. not as) the input image. We show that visual explanation, outperforming state of the art methods, can be derived from these. Our method is model-agnostic and, at test time, only requires a single forward pass to generate explanation. Therefore, the proposed approach is adapted for real-time systems such as medical image analysis. Finally, we show that random geometric augmentations applied on the original image acts as a regularization that improves all state of the art explanation methods. We validate our approach on a large chest X-ray database.